Innovation and Technology
The Rise of DEIA-Focused Job Boards: A Game-Changer for Inclusive Hiring
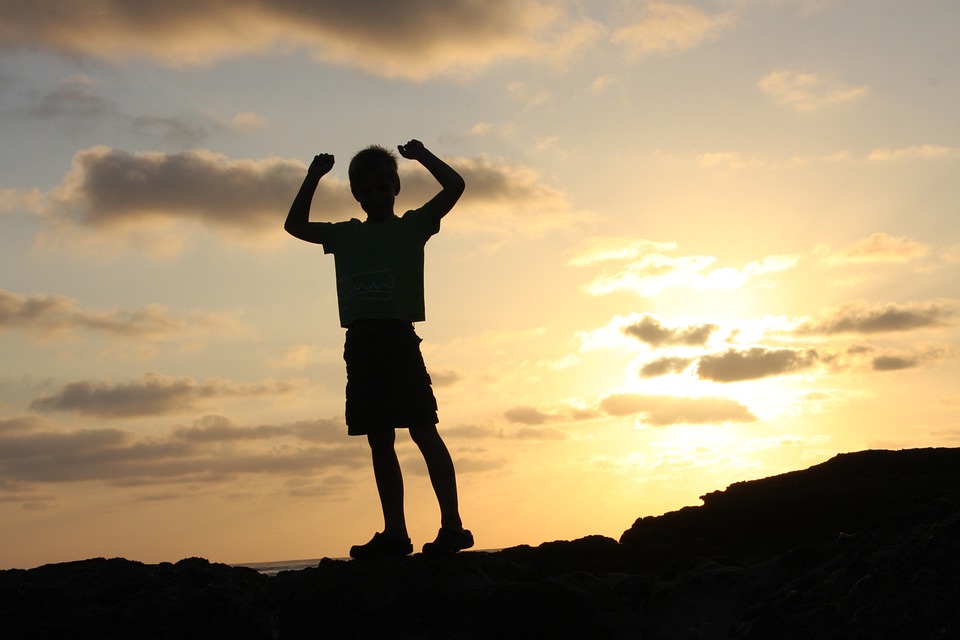
The world of recruitment has evolved significantly in recent years, with a growing emphasis on diversity, equity, and inclusion (DEIA). As companies strive to create a more diverse and inclusive workplace, DEIA-focused job boards have emerged as a game-changer for inclusive hiring. In this article, we’ll explore the rise of DEIA-focused job boards, their benefits, and how they’re revolutionizing the way we approach recruitment.
The Need for DEIA-Focused Job Boards
The importance of DEIA in the workplace cannot be overstated. A diverse and inclusive workplace is more productive, innovative, and better equipped to meet the needs of a diverse customer base. However, many organizations still struggle to attract and retain a diverse workforce. DEIA-focused job boards aim to address this issue by providing a platform for underrepresented groups to find job opportunities that cater to their needs.
What are DEIA-Focused Job Boards?
DEIA-focused job boards are online platforms that cater specifically to underrepresented groups, including women, people of color, individuals with disabilities, and members of the LGBTQ+ community. These platforms provide a safe and welcoming space for job seekers to search for job opportunities, network with other professionals, and access resources and support.
Benefits of DEIA-Focused Job Boards
1.
Increased Diversity and Inclusion
DEIA-focused job boards promote diversity and inclusion by providing a platform for underrepresented groups to access job opportunities. This helps to create a more diverse and inclusive workplace, which is critical for innovation and success.
2.
Improved Candidate Pool
By targeting specific communities, DEIA-focused job boards help organizations access a more diverse pool of candidates. This can lead to a more innovative and productive workforce, as well as better representation of the company’s target market.
3.
Enhanced Employer Branding
By showcasing their commitment to diversity and inclusion, companies that use DEIA-focused job boards can enhance their employer brand. This can lead to increased employee engagement, retention, and attraction of top talent.
4.
Cost-Effective
DEIA-focused job boards can be a cost-effective way to attract a diverse pool of candidates. By targeting specific communities, organizations can reduce the need for expensive recruitment agencies and job boards.
Examples of DEIA-Focused Job Boards
1.
Ellevation Collective
Ellevation Collective is a job board specifically designed for women in tech. The platform provides a safe and welcoming space for women to search for job opportunities, network with other professionals, and access resources and support.
2.
The Latinx in Tech Job Board
The Latinx in Tech Job Board is a platform that connects Latinx talent with job opportunities in the tech industry. The platform provides a space for Latinx professionals to showcase their skills and connect with other professionals in the industry.
3.
Disability:IN Job Board
The Disability:IN Job Board is a platform that connects people with disabilities with job opportunities. The platform provides a safe and welcoming space for individuals with disabilities to search for job opportunities, network with other professionals, and access resources and support.
Conclusion
The rise of DEIA-focused job boards is a game-changer for inclusive hiring. These platforms provide a safe and welcoming space for underrepresented groups to access job opportunities, network with other professionals, and access resources and support. By using DEIA-focused job boards, organizations can enhance their diversity and inclusion, access a more diverse pool of candidates, and improve their employer brand. As the world of recruitment continues to evolve, DEIA-focused job boards will play a critical role in creating a more inclusive and diverse workplace.
FAQs
Q: What is a DEIA-focused job board?
A: A DEIA-focused job board is an online platform that caters specifically to underrepresented groups, including women, people of color, individuals with disabilities, and members of the LGBTQ+ community.
Q: What are the benefits of using DEIA-focused job boards?
A: The benefits of using DEIA-focused job boards include increased diversity and inclusion, improved candidate pool, enhanced employer branding, and cost-effectiveness.
Q: What are some examples of DEIA-focused job boards?
A: Some examples of DEIA-focused job boards include Ellevation Collective, The Latinx in Tech Job Board, and the Disability:IN Job Board.
Q: How can organizations get started with DEIA-focused job boards?
A: Organizations can get started with DEIA-focused job boards by researching and selecting a platform that aligns with their diversity and inclusion goals. They should also provide training and support for their hiring managers on how to effectively use the platform.
Innovation and Technology
Get Better at Experimentation
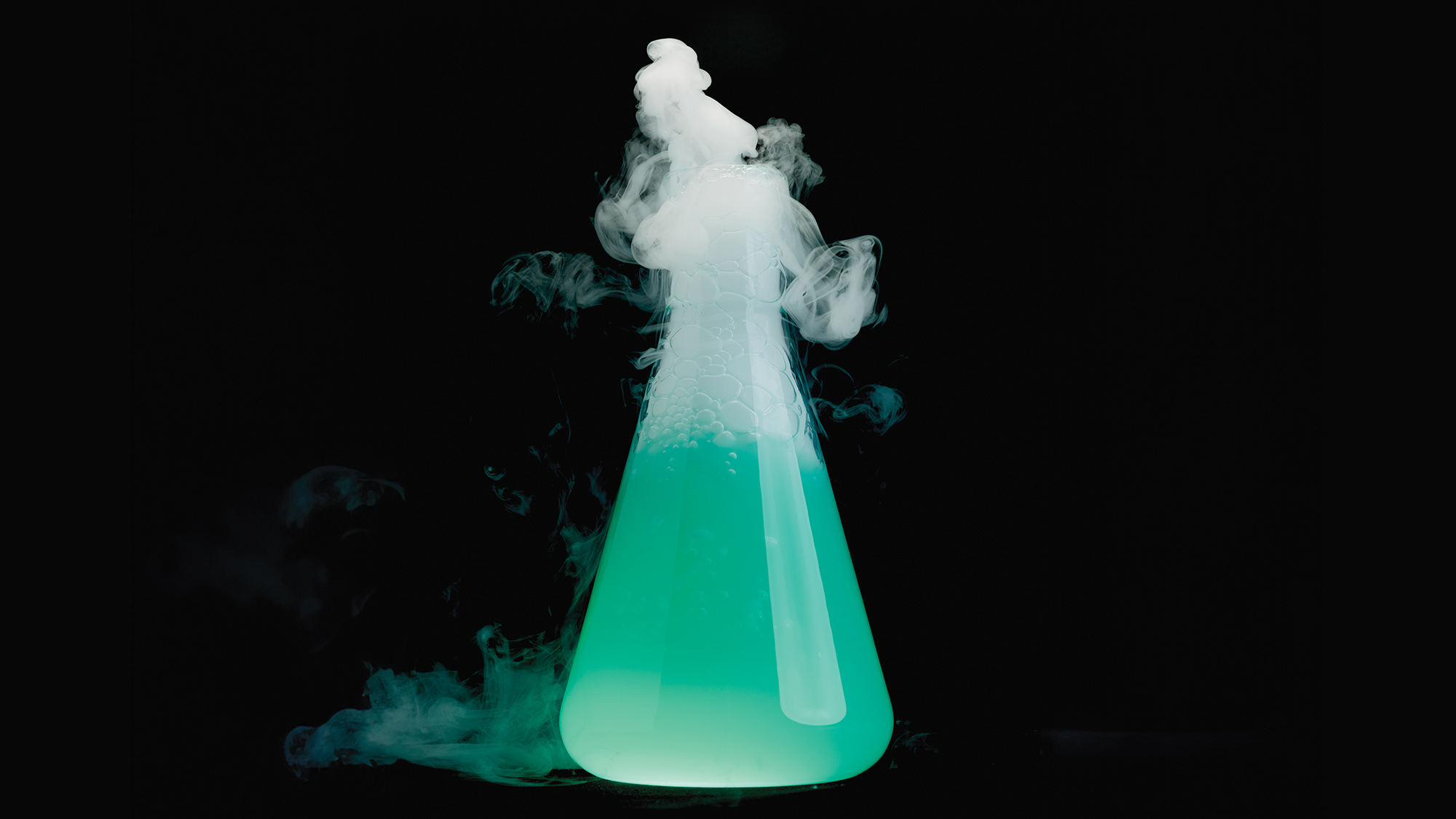
Want Your Company to Get Better at Experimentation?
Embracing Experimentation as a Culture
In today’s fast-paced and rapidly changing business environment, experimentation has become a crucial aspect of staying ahead of the competition. However, many companies struggle to make experimentation a part of their culture. To achieve success, experimentation must be ingrained in the organization’s DNA, and employees must be empowered to take calculated risks and learn from failures.
Why Experimentation is Crucial for Business Success
Experimentation allows companies to test new ideas, products, and services, and to learn from the results. It enables them to innovate, adapt to changing market conditions, and stay ahead of the competition. Moreover, experimentation helps to identify and mitigate risks, and to build a culture of continuous learning and improvement.
How to Encourage Experimentation in Your Company
1. Set Clear Goals and Objectives
To encourage experimentation, it is essential to set clear goals and objectives. This will help employees understand what they are trying to achieve and will provide a framework for experimentation. Goals should be specific, measurable, achievable, relevant, and time-bound (SMART).
2. Provide Resources and Support
To enable experimentation, companies must provide the necessary resources and support. This includes providing access to data, tools, and technology, as well as training and development opportunities.
3. Foster a Culture of Experimentation
Fostering a culture of experimentation requires creating an environment where employees feel comfortable taking calculated risks and learning from failures. This can be achieved by recognizing and rewarding experimentation, and by encouraging a growth mindset.
Best Practices for Experimentation
1. Define Your Experimentation Strategy
Before starting an experiment, it is essential to define the strategy and objectives. This will help to ensure that the experiment is focused and effective.
2. Design Your Experiment
The design of the experiment is critical to its success. It should be well-planned, and the variables should be carefully controlled.
3. Analyze and Learn from Results
After the experiment, it is essential to analyze the results and learn from them. This will help to identify what worked and what didn’t, and to inform future experiments.
Conclusion
In conclusion, experimentation is a crucial aspect of business success. To get better at experimentation, companies must embrace it as a culture, provide resources and support, and foster a culture of experimentation. By following best practices and learning from failures, companies can stay ahead of the competition and achieve long-term success.
FAQs
Q: What is experimentation in business?
A: Experimentation in business refers to the process of testing new ideas, products, and services, and learning from the results.
Q: Why is experimentation important for business success?
A: Experimentation is important for business success because it allows companies to innovate, adapt to changing market conditions, and stay ahead of the competition.
Q: How can I encourage experimentation in my company?
A: To encourage experimentation, set clear goals and objectives, provide resources and support, and foster a culture of experimentation.
Q: What are some best practices for experimentation?
A: Some best practices for experimentation include defining your experimentation strategy, designing your experiment, and analyzing and learning from results.
Innovation and Technology
5 Essential ChatGPT Prompts
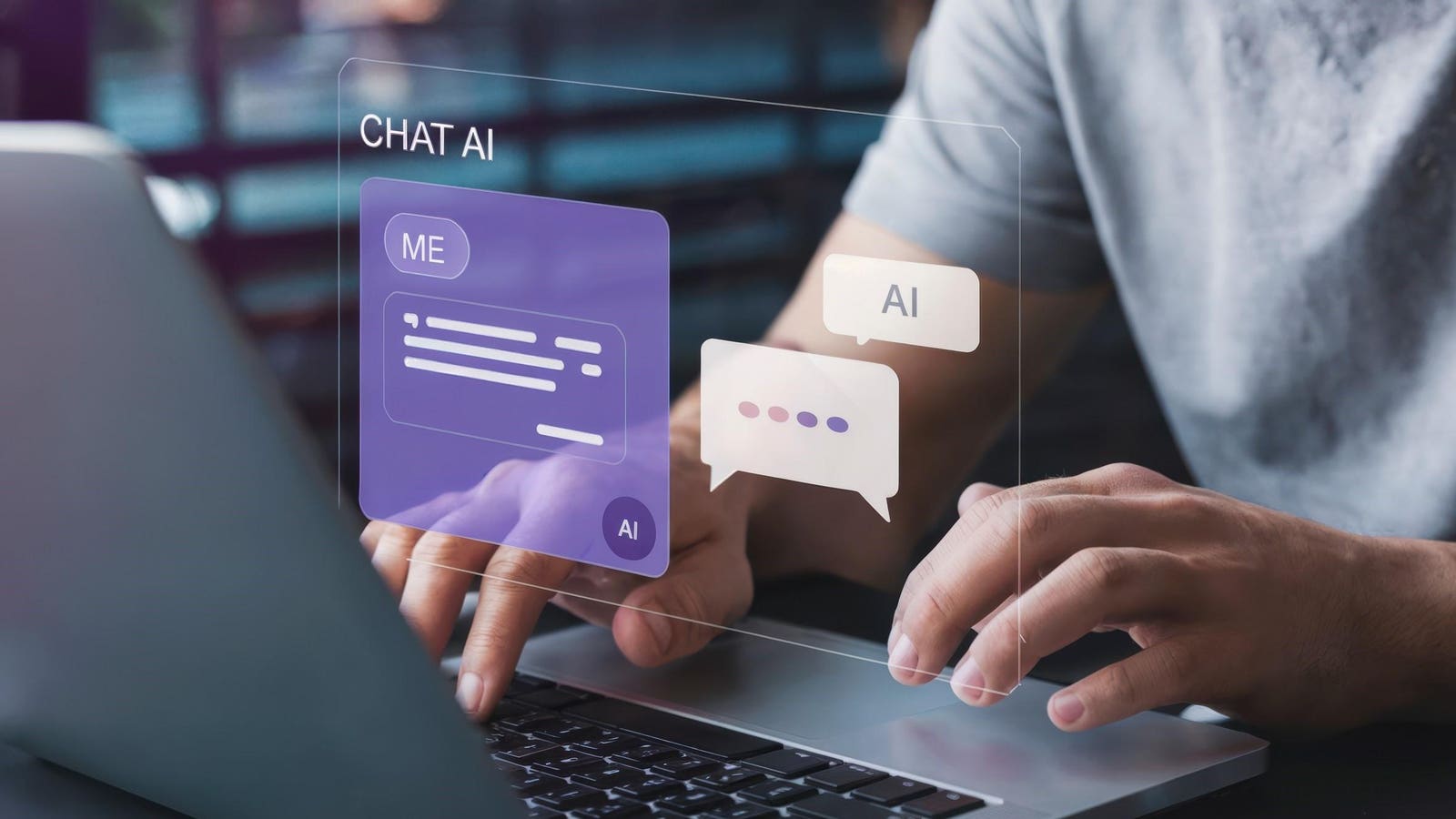
As Artificial Intelligence Reshapes the Workplace, HR Professionals and Recruiters Discover Powerful Ways to Leverage ChatGPT
The Secret to Effective Prompt Writing
From creating enticing job descriptions to quickly sifting and assessing candidates, there are many ways that ChatGPT and similar generative AI tools can help HR and recruiters work smarter. The secret lies in creating killer prompts – the instructions that tell chatbots and language models what we expect them to do. Just like when we’re asking humans to help us, the more precise we are, the more likely it is that the AI will know exactly what we want to do.
Prompt Writing: A Valuable Skill in HR and Recruitment
Prompt writing – sometimes called prompt engineering – is increasingly becoming a valuable skill in many areas of business. So, if you’re involved in HR or recruitment, here are some examples of prompts that can be used to help save time and cut repetitive work.
Conduct A Skills Gap Analysis
Identify areas where your organization may have to make new hires or upskill existing employees to meet its future needs:
I need your help to identify where there are skills gaps in our workforce that are preventing us from meeting our business goals. I will provide an overview of our strategic business priorities, as well as a skills assessment of our workforce and leadership teams. When you have enough data, use the information to generate a report highlighting areas where we should focus on recruitment or reskilling in order to achieve our objectives.
Write Great Job Descriptions
This prompt will take you through the steps of creating a job description that will attract candidates for any vacancy:
Act as a recruitment copywriter and help me write a job description optimized for generating applications. Start by asking me all the necessary details one question at a time (e.g., title, summary, location, responsibilities, skills, organization fit, growth opportunities, compensation, and benefits). Once you have enough information, craft a clear, professional, and engaging description using active language, bullet points, relevant keywords, and a strong call to action to improve search visibility.
Review Applicant’s CVs And Prepare Interview Questions
This prompt compares CVs against a job description, highlights how well they fit the role requirements and suggests questions to ask them at the interview. Be careful to comply with any data protection laws that apply to your business, for example, by removing personal data where necessary:
Please help me screen the CVs of job applicants. The job we are screening for is [insert job title], and this is the job description [paste job description]. I will upload CV files, and you will provide a summary of how each applicant matches up to the skills, experiences, and qualifications required for the role. Based on those insights, please also provide three questions I can ask the candidate in an interview to further assess their suitability.
Communicate A Company’s Enterprise Value Proposition
An Enterprise Value Proposition (EVP) outlines the benefits and opportunities that your company offers its employees. This prompt helps create material to let potential applicants know why it’s a great place to work:
Please help me create engaging EVP content for my company, [insert company name], which effectively and concisely communicates why it is a great place to work and is designed to help us be attractive to top talent. Ask questions, one at a time, to gather the information you need, and when you have enough, generate copy that can be used on our website, recruitment portals and social media channels.
Review Recruitment Processes To Identify And Remove Bias
This prompt helps identify and eliminate potential sources of bias in your hiring processes, ensuring your recruitment practices are fair and inclusive for all candidates:
Please act as a recruitment specialist and review our recruitment processes to ensure they are inclusive and free of bias. Ask me questions, one at a time, or ask me to provide information about any element of our recruitment processes that you need to know about in order to identify opportunities for bias to affect our recruitment outcomes. If you identify areas of concern in relation to the potential for bias, you can ask follow-up questions to clarify or ensure you have a full overview of the existing process. When you have enough information please provide an evaluation as well as advice on how we can further remove bias and achieve more inclusive outcomes.
Conclusion
As organizations continue to embrace AI tools in their HR and recruitment processes, the key to success lies in crafting effective prompts that align with your specific needs and objectives. By adapting and building upon these prompts, you can create a more efficient, inclusive, and strategic approach to talent acquisition and management. Remember that while AI is a powerful tool, it works best when combined with human insight and expertise.
FAQs
Q: What is prompt writing, and why is it important in HR and recruitment?
A: Prompt writing is the process of creating clear and specific instructions for AI tools to follow. It is important because it enables HR and recruitment professionals to get the most out of AI tools and achieve their goals more efficiently.
Q: How can I use ChatGPT to write great job descriptions?
A: You can use ChatGPT to write great job descriptions by providing it with the necessary details, such as job title, summary, location, responsibilities, skills, organization fit, growth opportunities, compensation, and benefits. The AI tool will then craft a clear, professional, and engaging description using active language, bullet points, relevant keywords, and a strong call to action.
Q: Can I use ChatGPT to review applicant’s CVs and prepare interview questions?
A: Yes, you can use ChatGPT to review applicant’s CVs and prepare interview questions. Simply provide the AI tool with the job description and CV files, and it will provide a summary of how each applicant matches up to the skills, experiences, and qualifications required for the role. It will also suggest questions to ask the candidate in an interview to further assess their suitability.
Innovation and Technology
Teams Get Locked Into Behavior Patterns
Like Siblings, Teams Get Locked Into Behavior Patterns
The Power of Habit
When we think about habits, we often think about individual behaviors, like brushing our teeth or checking our phones first thing in the morning. But what about team habits? Just like individuals, teams can develop patterns of behavior that become automatic and hard to break. These patterns can be both positive and negative, and they can have a significant impact on a team’s performance and success.
How Teams Develop Habits
So, how do teams develop these habits? It’s often a combination of factors, including:
- Shared experiences: Teams that go through similar challenges and successes together can develop a sense of camaraderie and shared understanding.
- Leadership: A team’s leader can influence the development of habits by setting an example, providing guidance, and reinforcing positive behaviors.
- Culture: A team’s culture can also play a role in shaping habits. For example, a team that values creativity and experimentation may develop habits that encourage innovation.
The Benefits of Positive Habits
Positive habits can have a significant impact on a team’s performance and success. For example:
- Improved communication: Teams that develop habits of open and honest communication can work more effectively together.
- Increased collaboration: Teams that develop habits of collaboration and teamwork can achieve more than they could alone.
- Better problem-solving: Teams that develop habits of creative problem-solving can find innovative solutions to complex challenges.
The Challenges of Negative Habits
Negative habits, on the other hand, can have a significant impact on a team’s performance and success. For example:
- Conflict: Teams that develop habits of conflict and competition can struggle to work together effectively.
- Burnout: Teams that develop habits of overwork and exhaustion can experience high levels of stress and burnout.
- Innovation stifling: Teams that develop habits of risk aversion and caution can struggle to innovate and adapt to changing circumstances.
Breaking Free from Negative Habits
So, how can teams break free from negative habits and develop positive ones? Here are a few strategies:
- Recognize and acknowledge: Recognize when a negative habit is developing and acknowledge its impact on the team.
- Identify the root cause: Identify the root cause of the negative habit and address it.
- Develop a new habit: Develop a new, positive habit to replace the old one.
- Practice and reinforce: Practice the new habit and reinforce it through positive feedback and recognition.
Conclusion
In conclusion, teams can develop habits just like individuals, and these habits can have a significant impact on a team’s performance and success. By recognizing and addressing negative habits and developing positive ones, teams can work more effectively together and achieve their goals.
FAQs
Q: How can I identify negative habits in my team?
A: Look for patterns of behavior that are causing problems or holding the team back. Ask team members to share their observations and concerns.
Q: How can I encourage positive habits in my team?
A: Lead by example, provide positive feedback and recognition, and create a culture that values collaboration and innovation.
Q: What if my team is stuck in a negative habit and can’t seem to break free?
A: Recognize that breaking a habit takes time and effort. Work with the team to identify the root cause of the habit and develop a plan to replace it with a positive one.
-
Career Advice1 month ago
Interview with Dr. Kristy K. Taylor, WORxK Global News Magazine Founder
-
Diversity and Inclusion (DEIA)1 month ago
Sarah Herrlinger Talks AirPods Pro Hearing Aid
-
Career Advice1 month ago
NetWork Your Way to Success: Top Tips for Maximizing Your Professional Network
-
Diversity and Inclusion (DEIA)1 month ago
The Power of Belonging: Why Feeling Accepted Matters in the Workplace
-
Global Trends and Politics1 month ago
Health-care stocks fall after Warren PBM bill, Brian Thompson shooting
-
Global Trends and Politics1 month ago
Unionization Goes Mainstream: How the Changing Workforce is Driving Demand for Collective Bargaining
-
Changemaker Interviews2 weeks ago
Unlocking Human Potential: Kim Groshek’s Journey to Transforming Leadership and Stress Resilience
-
Training and Development1 month ago
Level Up: How Upskilling Can Help You Stay Ahead of the Curve in a Rapidly Changing Industry