Innovation and Technology
The 5 Toughest Challenges On The Path To AGI
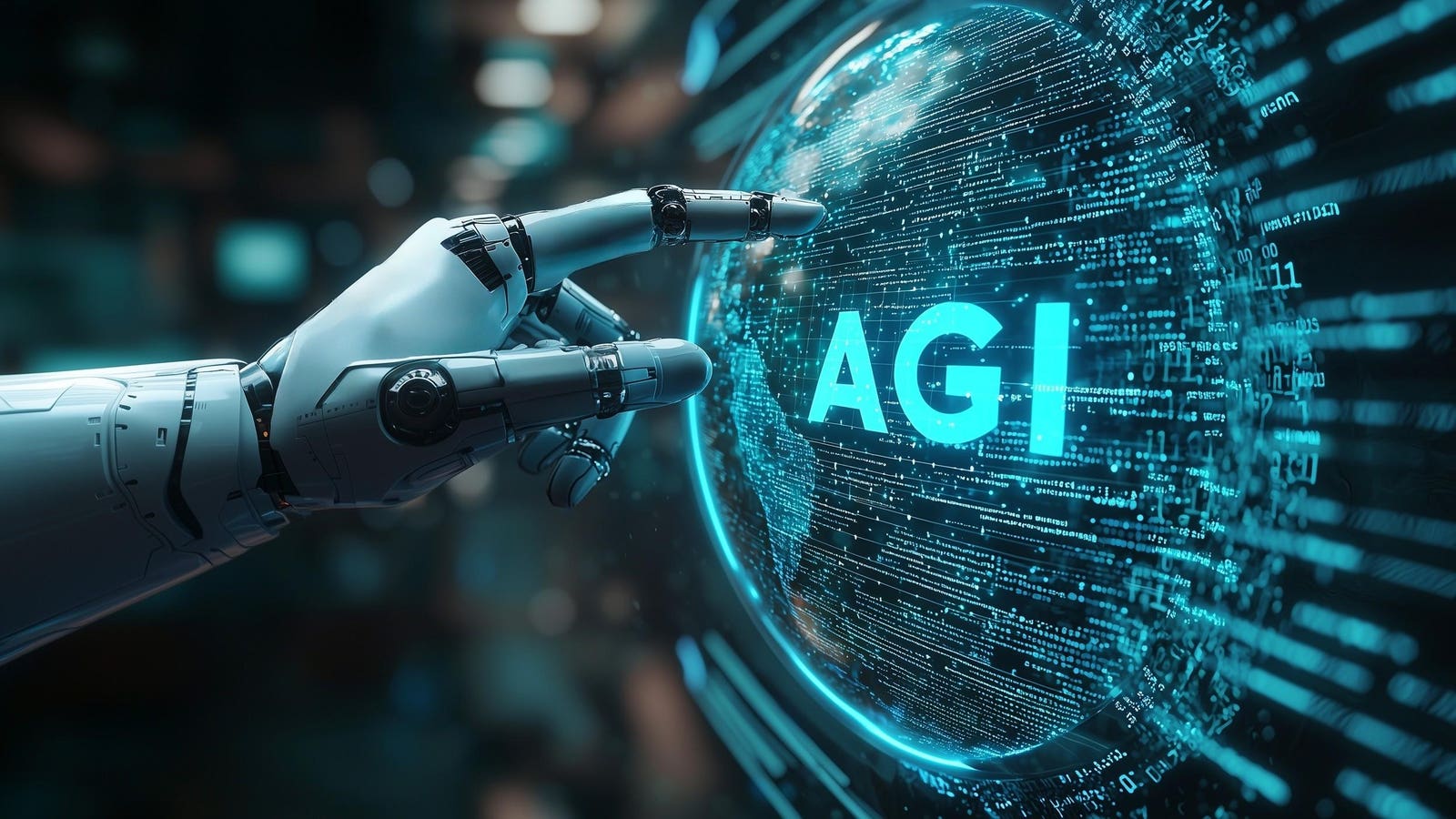
Innovation and Technology
AI’s Hidden Value
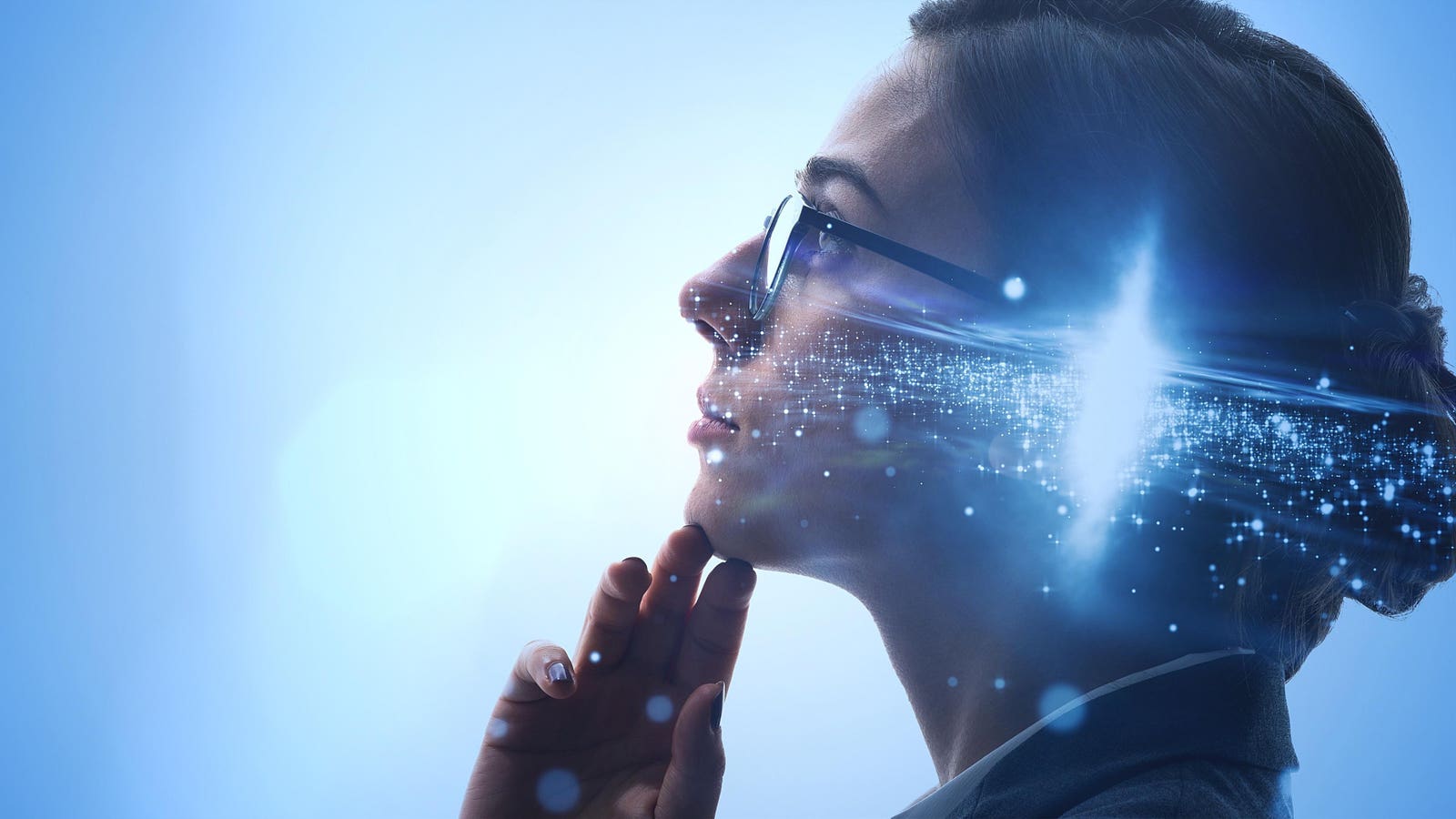
What the Future of AI Holds
The world of artificial intelligence (AI) and genAI is rapidly expanding, with numerous use cases emerging. While it’s easy to get caught up in the current applications of AI, such as customer personalization, data sorting, and productivity tools, it’s essential to look beyond the horizon and explore the potential innovations that may be on the way.
Autonomous Creativity: The Next Era of AI
According to Ben James, founder of 404, Bittensor Subnet 17, the next era of AI innovation will focus on autonomous creativity. "It will be about autonomous creativity," he said. "We can see a reality where AI does not just generate content but serves as architects of entire worlds that operate, think, evolve, and respond as dynamically to both human cues and AI-driven inputs." James believes that AI will be able to design persistent digital spaces that grow and thrive organically, following their own rules of governance, aesthetic, and cultural and operational dynamics.
Redefining Imagination and Creativity
This raises questions about the potential of AI to develop its own creative movements, rivaling the revolutions of the past. Will virtual worlds become intertwined and as culturally significant as ours? What happens when AI becomes the influence, and humans look to this technology for inspiration rather than the other way around? James predicts that humans and AI will collaborate as active teammates in the creative process, redefining what we know about imagination, creativity, and artistic collaboration.
AI-Enabled Communication and Prediction
Devansh Agarwal, senior machine learning engineer at Amazon Web Services, believes that AI may even help facilitate and predict human communication in new ways, via "interpreting wave-based signals." This includes waves like ultrasound waves, electromagnetic waves, or even WiFi. Agarwal explains that AI is being used to understand these waves, which could transform how day-to-day life happens. For example, imagine speaking without using your vocal cords, smart glasses that monitor if you’re chewing properly, or security systems detecting human presence and motion through Wi-Fi signals.
Decoding Wave Signals
Agarwal’s team at Cornell demonstrated the usage of ultrasound waves for predicting speech by analyzing silent mouth movements. This is possible because when waves interact with objects, they undergo phase shifts or amplitude changes, carrying rich information. With AI’s ability to analyze subtle variations, we can decode what caused these changes with unprecedented accuracy. Google’s project Soli initially broke ground in this area, employing a miniature radar chip that detects motion and understands nonverbal cues.
AI as an Early Warning System
Rita McGrath, professor at Columbia Business School, believes that AI can serve as an early warning system for a range of requirements, inside and outside. AI can conduct computations and run experiments that would be too unwieldy or expensive for people to calculate manually. For example, AI can look at early warnings of various kinds in arenas like public health, such as wastewater sampling for evidence of diseases like COVID-19.
Facilitating Experimentation
AI can facilitate experimentation on a very rapid scale in ways that could never happen in real life. For instance, Kraft-Heinz has built a digital twin of its whole supply chain – a virtual version of what they have in reality. Then, they can bring in a signal from the real world – say a supplier is going to be late, or there is a delay at the ports. What they can then do is simulate how the whole system might respond, running hundreds of simulations and having the system recommend the optimal response.
AI-Powered Synthetic Influencers
RTB Ruhan, CEO at Null Station, sees a future of "AI-powered synthetic influencers that fully run themselves." These would not simply be virtual characters created by a team but AI personalities that could learn, adapt, and build relationships on their own. Current virtual influencers, such as Lil Miquela, still rely on some form of human script and decision-making. However, Ruhan predicts that soon AI-driven personalities will be able to create content, negotiate brand deals, and even evolve their personalities through audience feedback.
The Future of Influencer Marketing
AI-powered influencers have many other talents as well. They would be able to fluently speak dozens of languages, personalize every interaction, and never miss a trend. For brands, this would be a major game-changer, as they would have AI personalities built from the ground up for their audience, scalable, always available, and fully malleable. Ruhan goes so far as to predict that people will become emotionally attached to these AI influencers, preferring them over human influencers because AI won’t have scandals, burnout, or inconsistency.
Conclusion
The future of AI holds much promise, from autonomous creativity to AI-enabled communication and prediction, early warning systems, and AI-powered synthetic influencers. As AI continues to evolve, we can expect to see significant advancements in various industries, transforming the way we live and interact with technology.
FAQs
- What is the next era of AI innovation?
The next era of AI innovation will focus on autonomous creativity, where AI serves as architects of entire worlds that operate, think, evolve, and respond as dynamically to both human cues and AI-driven inputs. - How can AI facilitate human communication?
AI can facilitate human communication by interpreting wave-based signals, such as ultrasound waves, electromagnetic waves, or WiFi, allowing for new forms of interaction, such as speaking without using vocal cords. - What is the role of AI in early warning systems?
AI can serve as an early warning system for a range of requirements, inside and outside, by conducting computations and running experiments that would be too unwieldy or expensive for people to calculate manually. - What are AI-powered synthetic influencers?
AI-powered synthetic influencers are AI personalities that can learn, adapt, and build relationships on their own, creating content, negotiating brand deals, and evolving their personalities through audience feedback. - How will AI-powered influencers change the marketing landscape?
AI-powered influencers will be able to fluently speak dozens of languages, personalize every interaction, and never miss a trend, making them a major game-changer for brands, who will have AI personalities built from the ground up for their audience, scalable, always available, and fully malleable.
Innovation and Technology
AI Agents Are Changing How We Shop—And Marketers Need to Catch Up
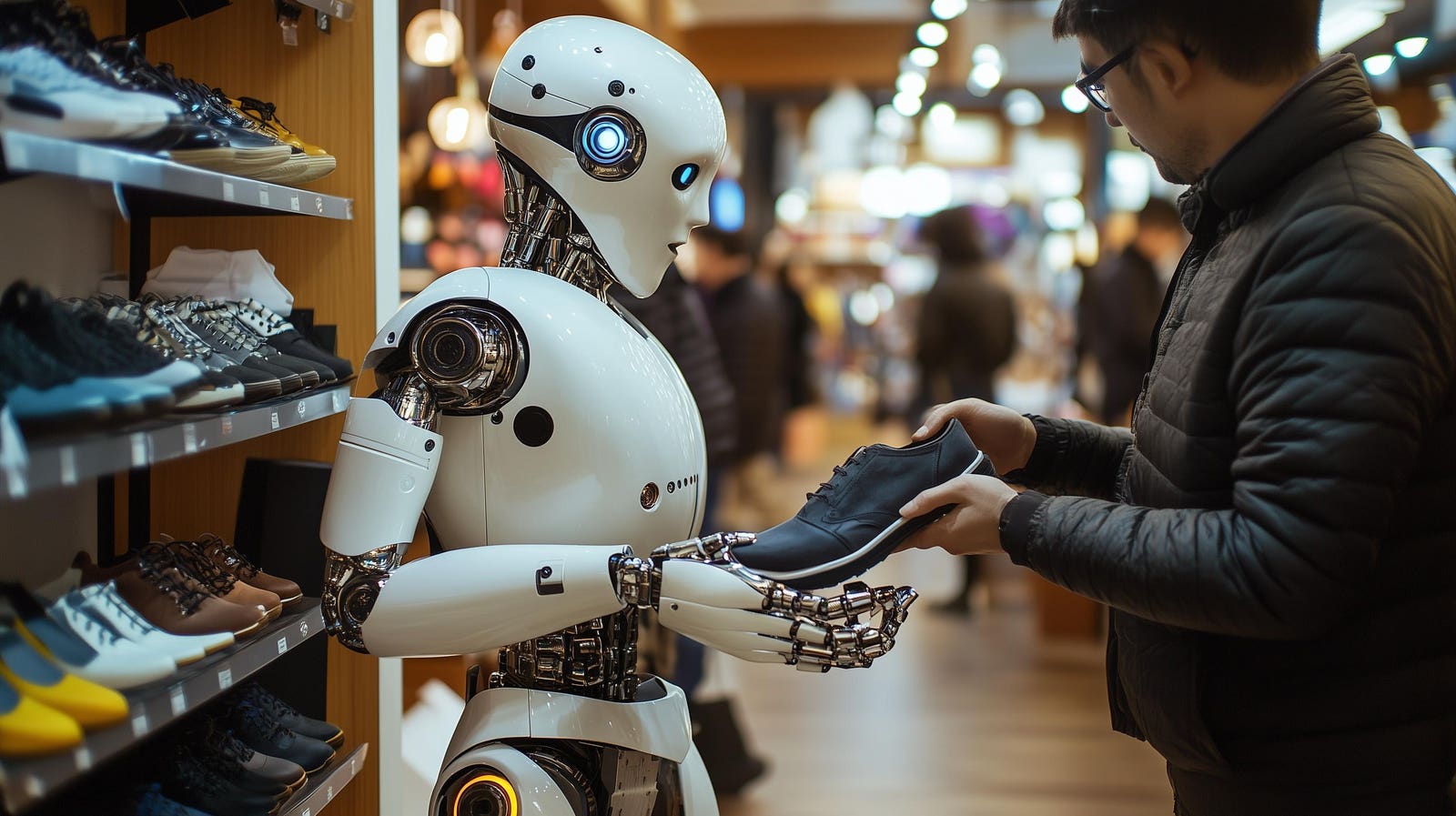
Shopping trends follow technology trends. Hot on the heels of each tech milestone of the digital age—the internet, social media, mobile—came marketers. Titans like Amazon, eBay and Temu were built by leveraging new opportunities to reach customers. The wave of transformation that will be driven by the widespread adoption of AI agents could potentially be even more dramatic. Agents are best thought of as the next leap forward in generative AI. Rather than simply thinking and talking like ChatGPT, agents are capable of taking action and performing complex tasks.
The Impact of AI Agents on Shopping
According to a recent research paper, Are AI Agents Interacting With Online Ads?, AI agents are already looking at advertising to decide whether it could help them answer questions. The paper also reports that the number of browser-based searches could drop by 25% by the end of this year in favor of searches carried out on our behalf by agentic or generative applications. So, what steps are businesses taking to prepare for this opportunity? And what does anyone responsible for marketing a business, from corporate marketers to small business owners, need to do to make sure they’re ready?
How Agents Shop And Make Buying Decisions
When explaining what agents can do, one of the most frequently cited examples is their ability to make buying decisions. By using a browser or interfacing APIs, they can, in theory, vastly speed up the process of making a purchase. While some of us might enjoy shopping, going through numerous sites to compare prices, shipping times, or return policies is still a time-consuming activity for humans. Agents are well suited to this because, with their analytical and language capabilities, they can quickly create an overview of our options, along with the pros and cons of choices, individually tailored to what they know about our needs.
Technical Capabilities of AI Agents
AI agents already exist that can help users by creating recipes to cook and shopping for ingredients, as well as scanning multiple sites looking for and booking the best travel deals, have already been demonstrated. The research examined behaviors of popular AI tools with agentic capabilities, including OpenAI Operator and open-source models. Among the findings was a propensity for agents to focus on structured data, such as lists of statistics, for the content they analyzed. It also discovered that agents using computer vision capabilities to “see” websites like us are easily tricked into clicking pop-ups.
How Can Businesses Leap On This Opportunity?
For marketers, this might seem to turn one of the fundamental principles of digital marketing on its head. Rather than persuading humans, the priority becomes persuading machines that are advising humans. The goal is to convince them that our ideas, messages, products or services are the best response to their users’ requests. The first clue we have is that we should focus on structured, machine-readable information. This might be because machines know we like information backed by facts and stats. But despite their ability to interpret complex expressive human languages, they still instinctively look for information in their native language of numbers.
Preparing for the Future of Marketing
The paper also suggests (although doesn’t definitively state) that well-sourced information with quality citations is more likely to attract the attention of agents. And it’s thought that agents are also more likely to be attracted to information that appears to be direct answers to questions, written in a clear and well-structured way. On the technical side, it would be a good idea to see if APIs and feeds can (and should) be made agent-friendly, opening up streams of data on products, prices or availability. This might involve marketers working with digital or e-commerce teams to make sure everyone’s on the same page.
Conclusion
It’s very hard to predict how any hugely transformative technology will work out long-term, and agentic AI is no different. When marketers started SEOing content decades ago, it was largely a technical exercise to count keywords. Today, it’s a vast and complex field of research, theory and practice involving many aspects of human and AI communication. Understanding the difference between human and machine decision-making will be the key here. How will the way that agents apply weight to factors like value, brand recognition or safety differ from the way we do? The most important piece of advice is to avoid the temptation to wait and see. The revolution is already underway, and as a growing number of AI service providers begin offering agentic platforms and apps, it could quickly become part of everyday life.
FAQs
- Q: What are AI agents and how do they work?
A: AI agents are advanced forms of generative AI that can perform complex tasks and make decisions on behalf of users. - Q: How will AI agents change the way we shop online?
A: AI agents are expected to reduce the number of browser-based searches by 25% by the end of the year, as they take over tasks such as comparing prices and finding the best deals. - Q: What can businesses do to prepare for the rise of AI agents in marketing?
A: Businesses should focus on creating structured, machine-readable information and making their APIs and feeds agent-friendly to attract the attention of AI agents. - Q: Will traditional SEO still be relevant in the age of AI agents?
A: While AI agents will change the way marketing works, traditional SEO will still be important, but businesses should also explore new ways to attract the attention of agentic AI.
Innovation and Technology
How AI-Powered Analytics Can Enhance Your Digital Transformation Journey
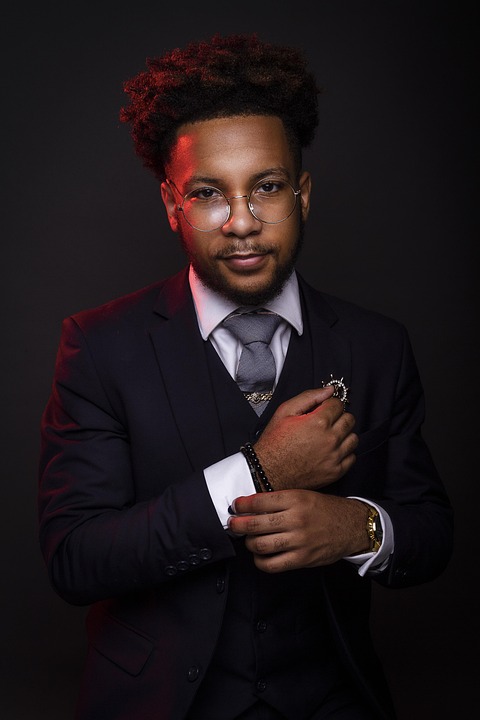
As organizations embark on digital transformation strategies, they are constantly seeking innovative ways to optimize their operations and gain a competitive edge. One crucial aspect of this journey is the implementation of predictive maintenance, a approach that leverages AI-powered analytics to forecast equipment failures and reduce downtime. By harnessing the power of data and machine learning, businesses can streamline their maintenance processes, minimize costs, and maximize productivity.
Understanding Predictive Maintenance
Predictive maintenance is a proactive approach to maintenance that uses advanced analytics and machine learning algorithms to predict when equipment is likely to fail or require maintenance. This approach enables organizations to schedule maintenance activities during planned downtime, reducing the likelihood of unexpected failures and minimizing the impact on operations. By analyzing data from various sources, including sensors, logs, and maintenance records, predictive maintenance systems can identify patterns and anomalies that may indicate potential issues.
Benefits of Predictive Maintenance
The benefits of predictive maintenance are numerous, and organizations that have implemented this approach have seen significant improvements in their operations. Some of the key benefits include reduced downtime, increased productivity, and lower maintenance costs. By predicting and preventing equipment failures, organizations can minimize the impact of downtime on their operations, reduce the need for costly repairs, and extend the lifespan of their assets.
How Predictive Maintenance Works
Predictive maintenance works by analyzing data from various sources, including sensors, logs, and maintenance records. This data is then fed into machine learning algorithms that identify patterns and anomalies that may indicate potential issues. The algorithms can detect subtle changes in equipment performance, such as changes in temperature, vibration, or pressure, that may indicate a potential problem. By analyzing this data, predictive maintenance systems can predict when equipment is likely to fail or require maintenance, enabling organizations to schedule maintenance activities during planned downtime.
AI-Powered Analytics for Predictive Maintenance
AI-powered analytics play a crucial role in predictive maintenance, enabling organizations to analyze large amounts of data and identify patterns and anomalies that may indicate potential issues. Machine learning algorithms can be trained on historical data to identify relationships between different variables, such as equipment performance, maintenance activities, and environmental factors. By analyzing this data, AI-powered analytics can predict when equipment is likely to fail or require maintenance, enabling organizations to take proactive measures to prevent downtime.
Machine Learning Algorithms for Predictive Maintenance
Several machine learning algorithms can be used for predictive maintenance, including supervised learning, unsupervised learning, and reinforcement learning. Supervised learning algorithms can be trained on labeled data to predict equipment failures, while unsupervised learning algorithms can identify patterns and anomalies in unlabeled data. Reinforcement learning algorithms can learn from trial and error, enabling them to optimize maintenance strategies over time.
Real-Time Data Analytics for Predictive Maintenance
Real-time data analytics is critical for predictive maintenance, enabling organizations to analyze data as it is generated and respond quickly to potential issues. By analyzing real-time data from sensors and other sources, organizations can identify changes in equipment performance and take proactive measures to prevent downtime. Real-time data analytics can also enable organizations to optimize their maintenance strategies, scheduling maintenance activities during planned downtime and minimizing the impact on operations.
Implementation of Predictive Maintenance
Implementing predictive maintenance requires a structured approach, starting with the collection and analysis of data. Organizations must identify the equipment and assets that are critical to their operations and collect data on their performance, maintenance activities, and environmental factors. This data must then be analyzed using machine learning algorithms to identify patterns and anomalies that may indicate potential issues.
Data Collection and Integration
Data collection and integration are critical components of predictive maintenance, enabling organizations to analyze data from various sources and identify patterns and anomalies. Organizations must collect data from sensors, logs, and maintenance records, and integrate it into a single platform for analysis. This data must be accurate, complete, and consistent, enabling organizations to make informed decisions about maintenance activities.
Change Management and Training
Change management and training are essential for the successful implementation of predictive maintenance. Organizations must communicate the benefits of predictive maintenance to stakeholders, including maintenance personnel, operators, and management. Training programs must be developed to educate personnel on the use of predictive maintenance systems, enabling them to analyze data and make informed decisions about maintenance activities.
Conclusion
Predictive maintenance is a critical component of digital transformation strategies, enabling organizations to optimize their operations, reduce downtime, and increase productivity. By leveraging AI-powered analytics and machine learning algorithms, organizations can predict equipment failures and schedule maintenance activities during planned downtime. The implementation of predictive maintenance requires a structured approach, starting with the collection and analysis of data, and including change management and training. By adopting predictive maintenance, organizations can gain a competitive edge, reduce costs, and maximize their returns on investment.
Frequently Asked Questions (FAQs)
Q: What is predictive maintenance?
A: Predictive maintenance is a proactive approach to maintenance that uses advanced analytics and machine learning algorithms to predict when equipment is likely to fail or require maintenance.
Q: What are the benefits of predictive maintenance?
A: The benefits of predictive maintenance include reduced downtime, increased productivity, and lower maintenance costs.
Q: How does predictive maintenance work?
A: Predictive maintenance works by analyzing data from various sources, including sensors, logs, and maintenance records, and using machine learning algorithms to identify patterns and anomalies that may indicate potential issues.
Q: What is the role of AI-powered analytics in predictive maintenance?
A: AI-powered analytics play a crucial role in predictive maintenance, enabling organizations to analyze large amounts of data and identify patterns and anomalies that may indicate potential issues.
Q: What are the common machine learning algorithms used in predictive maintenance?
A: Common machine learning algorithms used in predictive maintenance include supervised learning, unsupervised learning, and reinforcement learning.
-
Career Advice5 months ago
Interview with Dr. Kristy K. Taylor, WORxK Global News Magazine Founder
-
Diversity and Inclusion (DEIA)5 months ago
Sarah Herrlinger Talks AirPods Pro Hearing Aid
-
Career Advice5 months ago
NetWork Your Way to Success: Top Tips for Maximizing Your Professional Network
-
Changemaker Interviews4 months ago
Unlocking Human Potential: Kim Groshek’s Journey to Transforming Leadership and Stress Resilience
-
Diversity and Inclusion (DEIA)5 months ago
The Power of Belonging: Why Feeling Accepted Matters in the Workplace
-
Global Trends and Politics5 months ago
Health-care stocks fall after Warren PBM bill, Brian Thompson shooting
-
Global Trends and Politics5 months ago
Unionization Goes Mainstream: How the Changing Workforce is Driving Demand for Collective Bargaining
-
Training and Development5 months ago
Level Up: How Upskilling Can Help You Stay Ahead of the Curve in a Rapidly Changing Industry