Innovation and Technology
Big AI Inference Has Become a Big Deal and a Bigger Business
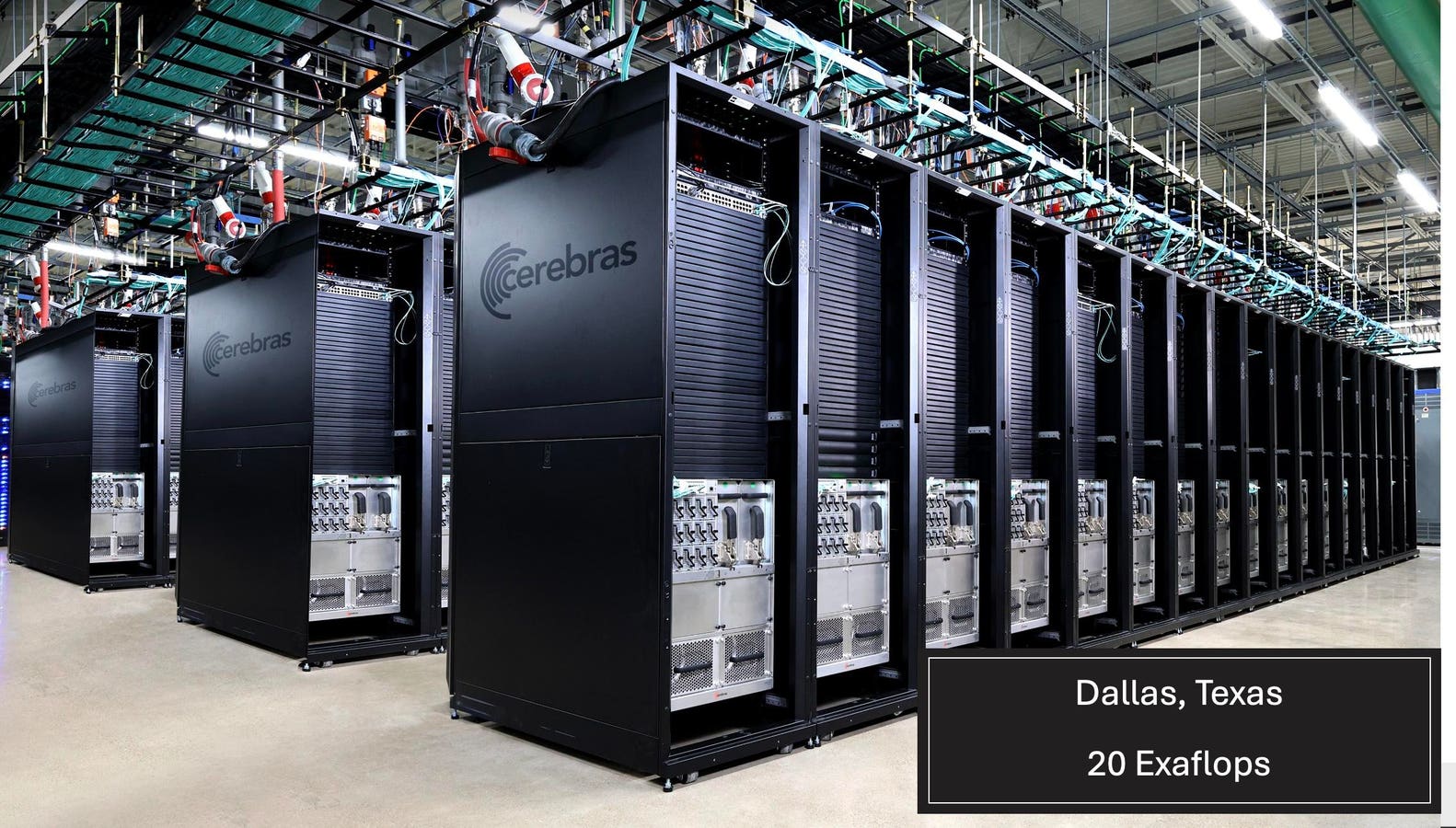
Cerebras Takes Inference To a New Level
Cerebras Systems, the creator of wafer-scale, Frisbee-sized AI chips, has rolled out a plan to build six new data centers since entering the “high-value” token business. The company claims it will become the largest provider of such inference services globally by the end of this year. The new data centers are partially up and running today and will soon expand to France and Canada. The aggregate capacity of these systems, which will number in the thousands, will exceed 40 million Llama 70B tokens per second.
High-Value Tokens
High-value tokens carry more contextual information and are typically more important for understanding the overall meaning of a text. They often represent key concepts, rare words, or specialized terminology. High-value tokens consume more computational resources and may cost more to process. This is because they typically require more attention from the model and contribute more significantly to the final output. Low-value tokens, which are more common and less informationally dense, usually require fewer processing resources. Clearly, Cerebras is targeting problems that are a good fit for its wafer-scale approach to AI.
The Inference Revolution is Just Beginning
Next week, we will hear more about “high-value” tokens from Nvidia at GTC, as the inference market overtakes training in global revenue. Markets such as autonomous vehicles, robots, and sovereign data centers all depend on fast inference, and Nvidia does not plan to let that market pass them by. The high-value concept is new, and platforms like Cerebras and Nvidia LVL72 are ideal for delivering it.
Achieving High-Performance Inference
Cerebras is 30 times faster and 90% cheaper due to its wafer-scale architecture. This level of performance in delivering high-value tokens is attracting new enterprise customers that also need elastic services to meet their needs. AlphaSense, for example, a leading market intelligence platform, has moved to Cerebras Inference, replacing a top-three closed-source AI model provider. The company has also landed Perplexity, Mistral, Hugging Face, and other users of high-value inferencing, delivering inference performance 10 to 20 times faster than alternatives.
Conclusion
Cerebras’ recent announcement marks a significant milestone in the development of AI inference technology. With its wafer-scale architecture, Cerebras is poised to become the largest provider of inference services globally by the end of the year. As the inference market continues to grow, we can expect to see more innovations and advancements in this space. Cerebras’ focus on high-value tokens and its ability to deliver fast and efficient inference services make it an attractive option for enterprises looking to leverage AI for their business needs.
FAQs
What is Cerebras Systems? Cerebras Systems is the creator of wafer-scale, Frisbee-sized AI chips.
What is high-value token? High-value tokens carry more contextual information and are typically more important for understanding the overall meaning of a text.
How does Cerebras achieve high-performance inference? Cerebras is 30 times faster and 90% cheaper due to its wafer-scale architecture.
What is the significance of the inference market? The inference market is expected to surpass the training market in global revenue, with applications in autonomous vehicles, robots, and sovereign data centers, among others.
Who are Cerebras’ clients? Cerebras’ clients include Baya Systems, BrainChip, Cadence, Cerebras Systems, D-Matrix, Esperanto, Flex, Groq, IBM, Intel, Micron, NVIDIA, Qualcomm, Graphcore, SImA.ai, Synopsys, Tenstorrent, Ventana Microsystems, and scores of investors.
Innovation and Technology
Marketing Revolution
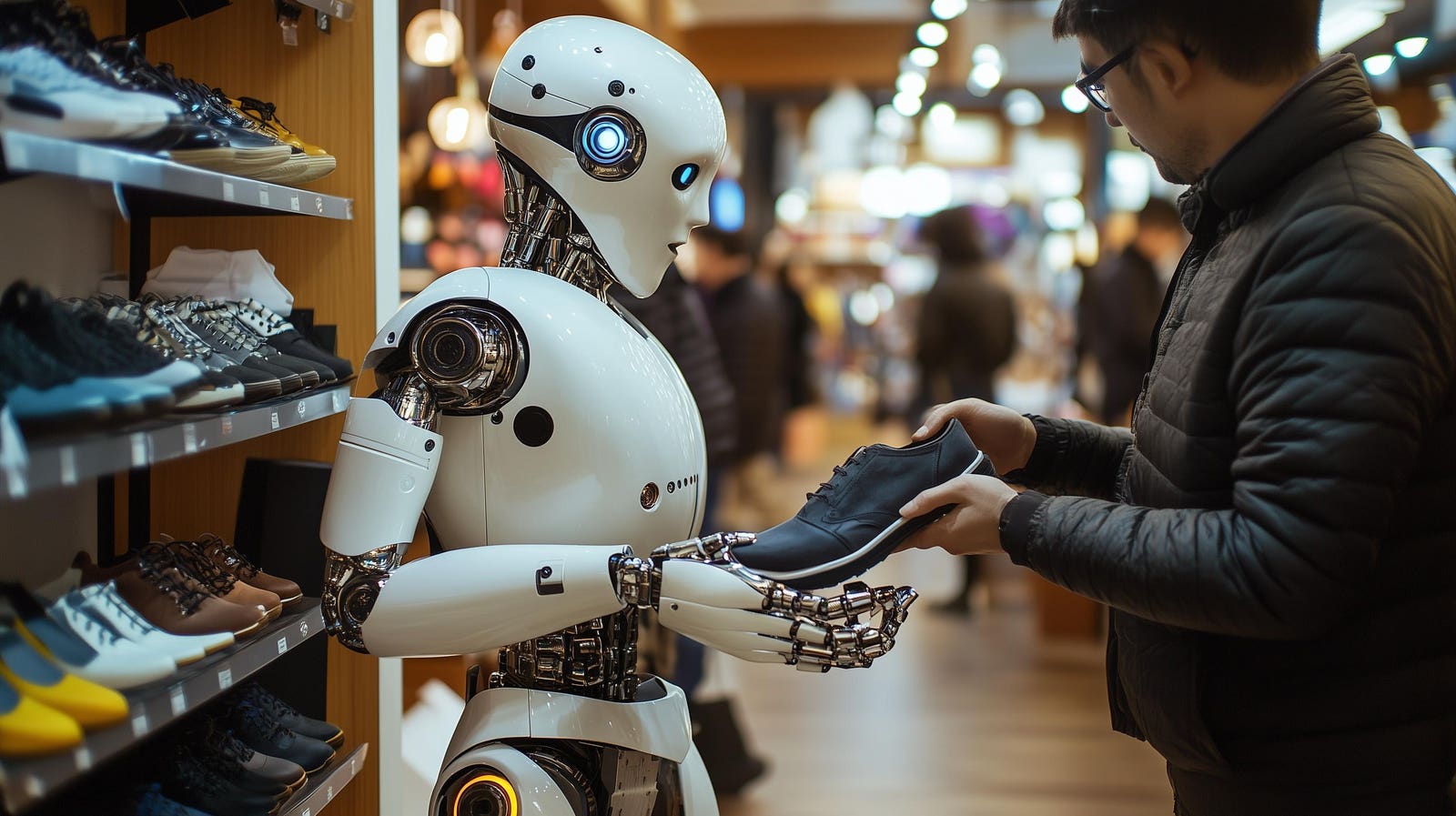
Introduction to AI Agents in Shopping
Shopping trends follow technology trends. Hot on the heels of each tech milestone of the digital age—the internet, social media, mobile—came marketers. Titans like Amazon, eBay and Temu were built by leveraging new opportunities to reach customers. The wave of transformation that will be driven by the widespread adoption of AI agents could potentially be even more dramatic. Agents are best thought of as the next leap forward in generative AI. Rather than simply thinking and talking like ChatGPT, agents are capable of taking action and performing complex tasks.
The Impact of AI Agents on Shopping
According to a recent research paper, Are AI Agents Interacting With Online Ads?, AI agents are already looking at advertising to decide whether it could help them answer questions. The paper also reports that the number of browser-based searches could drop by 25% by the end of this year in favor of searches carried out on our behalf by agentic or generative applications. So, what steps are businesses taking to prepare for this opportunity? And what does anyone responsible for marketing a business, from corporate marketers to small business owners, need to do to make sure they’re ready?
How Agents Shop And Make Buying Decisions
When explaining what agents can do, one of the most frequently cited examples is their ability to make buying decisions. By using a browser or interfacing APIs, they can, in theory, vastly speed up the process of making a purchase. While some of us might enjoy shopping, going through numerous sites to compare prices, shipping times, or return policies is still a time-consuming activity for humans. Agents are well suited to this because, with their analytical and language capabilities, they can quickly create an overview of our options, along with the pros and cons of choices, individually tailored to what they know about our needs.
Technical Capabilities of AI Agents
AI agents already exist that can help users by creating recipes to cook and shopping for ingredients, as well as scanning multiple sites looking for and booking the best travel deals, have already been demonstrated. The research examined behaviors of popular AI tools with agentic capabilities, including OpenAI Operator and open-source models. Among the findings was a propensity for agents to focus on structured data, such as lists of statistics, for the content they analyzed. It also discovered that agents using computer vision capabilities to “see” websites like us are easily tricked into clicking pop-ups.
How Can Businesses Leap On This Opportunity?
For marketers, this might seem to turn one of the fundamental principles of digital marketing on its head. Rather than persuading humans, the priority becomes persuading machines that are advising humans. The goal is to convince them that our ideas, messages, products or services are the best response to their users’ requests. The first clue we have is that we should focus on structured, machine-readable information. This might be because machines know we like information backed by facts and stats. But despite their ability to interpret complex expressive human languages, they still instinctively look for information in their native language of numbers.
Preparing for the Future of Marketing
The paper also suggests (although doesn’t definitively state) that well-sourced information with quality citations is more likely to attract the attention of agents. And it’s thought that agents are also more likely to be attracted to information that appears to be direct answers to questions, written in a clear and well-structured way. On the technical side, it would be a good idea to see if APIs and feeds can (and should) be made agent-friendly, opening up streams of data on products, prices or availability. This might involve marketers working with digital or e-commerce teams to make sure everyone’s on the same page.
Conclusion
It’s very hard to predict how any hugely transformative technology will work out long-term, and agentic AI is no different. When marketers started SEOing content decades ago, it was largely a technical exercise to count keywords. Today, it’s a vast and complex field of research, theory and practice involving many aspects of human and AI communication. Understanding the difference between human and machine decision-making will be the key here. How will the way that agents apply weight to factors like value, brand recognition or safety differ from the way we do? The most important piece of advice is to avoid the temptation to wait and see. The revolution is already underway, and as a growing number of AI service providers begin offering agentic platforms and apps, it could quickly become part of everyday life.
FAQs
- Q: What are AI agents and how do they work?
A: AI agents are advanced forms of generative AI that can perform complex tasks and make decisions on behalf of users. - Q: How will AI agents change the way we shop online?
A: AI agents are expected to reduce the number of browser-based searches by 25% by the end of the year, as they take over tasks such as comparing prices and finding the best deals. - Q: What can businesses do to prepare for the rise of AI agents in marketing?
A: Businesses should focus on creating structured, machine-readable information and making their APIs and feeds agent-friendly to attract the attention of AI agents. - Q: Will traditional SEO still be relevant in the age of AI agents?
A: While AI agents will change the way marketing works, traditional SEO will still be important, but businesses should also explore new ways to attract the attention of agentic AI.
Innovation and Technology
Predictive Maintenance: How AI-Powered Analytics Can Enhance Your Digital Transformation Journey
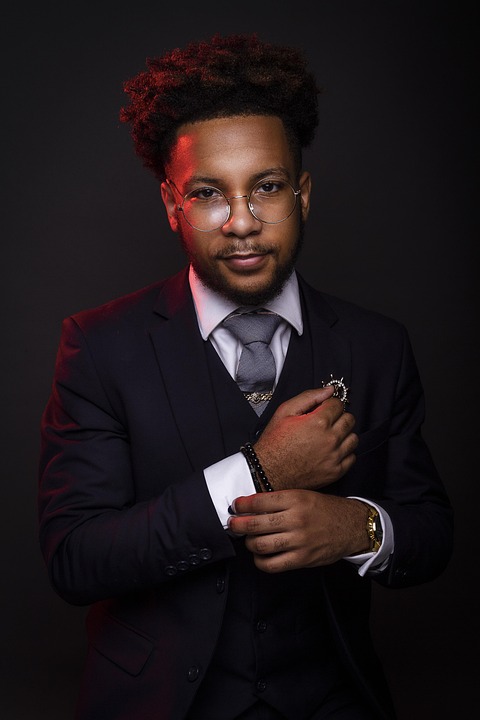
As organizations embark on digital transformation strategies, they are constantly seeking innovative ways to optimize their operations and gain a competitive edge. One crucial aspect of this journey is the implementation of predictive maintenance, a approach that leverages AI-powered analytics to forecast equipment failures and reduce downtime. By harnessing the power of data and machine learning, businesses can streamline their maintenance processes, minimize costs, and maximize productivity.
Understanding Predictive Maintenance
Predictive maintenance is a proactive approach to maintenance that uses advanced analytics and machine learning algorithms to predict when equipment is likely to fail or require maintenance. This approach enables organizations to schedule maintenance activities during planned downtime, reducing the likelihood of unexpected failures and minimizing the impact on operations. By analyzing data from various sources, including sensors, logs, and maintenance records, predictive maintenance systems can identify patterns and anomalies that may indicate potential issues.
Benefits of Predictive Maintenance
The benefits of predictive maintenance are numerous, and organizations that have implemented this approach have seen significant improvements in their operations. Some of the key benefits include reduced downtime, increased productivity, and lower maintenance costs. By predicting and preventing equipment failures, organizations can minimize the impact of downtime on their operations, reduce the need for costly repairs, and extend the lifespan of their assets.
How Predictive Maintenance Works
Predictive maintenance works by analyzing data from various sources, including sensors, logs, and maintenance records. This data is then fed into machine learning algorithms that identify patterns and anomalies that may indicate potential issues. The algorithms can detect subtle changes in equipment performance, such as changes in temperature, vibration, or pressure, that may indicate a potential problem. By analyzing this data, predictive maintenance systems can predict when equipment is likely to fail or require maintenance, enabling organizations to schedule maintenance activities during planned downtime.
AI-Powered Analytics for Predictive Maintenance
AI-powered analytics play a crucial role in predictive maintenance, enabling organizations to analyze large amounts of data and identify patterns and anomalies that may indicate potential issues. Machine learning algorithms can be trained on historical data to identify relationships between different variables, such as equipment performance, maintenance activities, and environmental factors. By analyzing this data, AI-powered analytics can predict when equipment is likely to fail or require maintenance, enabling organizations to take proactive measures to prevent downtime.
Machine Learning Algorithms for Predictive Maintenance
Several machine learning algorithms can be used for predictive maintenance, including supervised learning, unsupervised learning, and reinforcement learning. Supervised learning algorithms can be trained on labeled data to predict equipment failures, while unsupervised learning algorithms can identify patterns and anomalies in unlabeled data. Reinforcement learning algorithms can learn from trial and error, enabling them to optimize maintenance strategies over time.
Real-Time Data Analytics for Predictive Maintenance
Real-time data analytics is critical for predictive maintenance, enabling organizations to analyze data as it is generated and respond quickly to potential issues. By analyzing real-time data from sensors and other sources, organizations can identify changes in equipment performance and take proactive measures to prevent downtime. Real-time data analytics can also enable organizations to optimize their maintenance strategies, scheduling maintenance activities during planned downtime and minimizing the impact on operations.
Implementation of Predictive Maintenance
Implementing predictive maintenance requires a structured approach, starting with the collection and analysis of data. Organizations must identify the equipment and assets that are critical to their operations and collect data on their performance, maintenance activities, and environmental factors. This data must then be analyzed using machine learning algorithms to identify patterns and anomalies that may indicate potential issues.
Data Collection and Integration
Data collection and integration are critical components of predictive maintenance, enabling organizations to analyze data from various sources and identify patterns and anomalies. Organizations must collect data from sensors, logs, and maintenance records, and integrate it into a single platform for analysis. This data must be accurate, complete, and consistent, enabling organizations to make informed decisions about maintenance activities.
Change Management and Training
Change management and training are essential for the successful implementation of predictive maintenance. Organizations must communicate the benefits of predictive maintenance to stakeholders, including maintenance personnel, operators, and management. Training programs must be developed to educate personnel on the use of predictive maintenance systems, enabling them to analyze data and make informed decisions about maintenance activities.
Conclusion
Predictive maintenance is a critical component of digital transformation strategies, enabling organizations to optimize their operations, reduce downtime, and increase productivity. By leveraging AI-powered analytics and machine learning algorithms, organizations can predict equipment failures and schedule maintenance activities during planned downtime. The implementation of predictive maintenance requires a structured approach, starting with the collection and analysis of data, and including change management and training. By adopting predictive maintenance, organizations can gain a competitive edge, reduce costs, and maximize their returns on investment.
Frequently Asked Questions (FAQs)
Q: What is predictive maintenance?
A: Predictive maintenance is a proactive approach to maintenance that uses advanced analytics and machine learning algorithms to predict when equipment is likely to fail or require maintenance.
Q: What are the benefits of predictive maintenance?
A: The benefits of predictive maintenance include reduced downtime, increased productivity, and lower maintenance costs.
Q: How does predictive maintenance work?
A: Predictive maintenance works by analyzing data from various sources, including sensors, logs, and maintenance records, and using machine learning algorithms to identify patterns and anomalies that may indicate potential issues.
Q: What is the role of AI-powered analytics in predictive maintenance?
A: AI-powered analytics play a crucial role in predictive maintenance, enabling organizations to analyze large amounts of data and identify patterns and anomalies that may indicate potential issues.
Q: What are the common machine learning algorithms used in predictive maintenance?
A: Common machine learning algorithms used in predictive maintenance include supervised learning, unsupervised learning, and reinforcement learning.
Innovation and Technology
Why Business Leaders Are Losing Trust in Data—And What It Means for AI
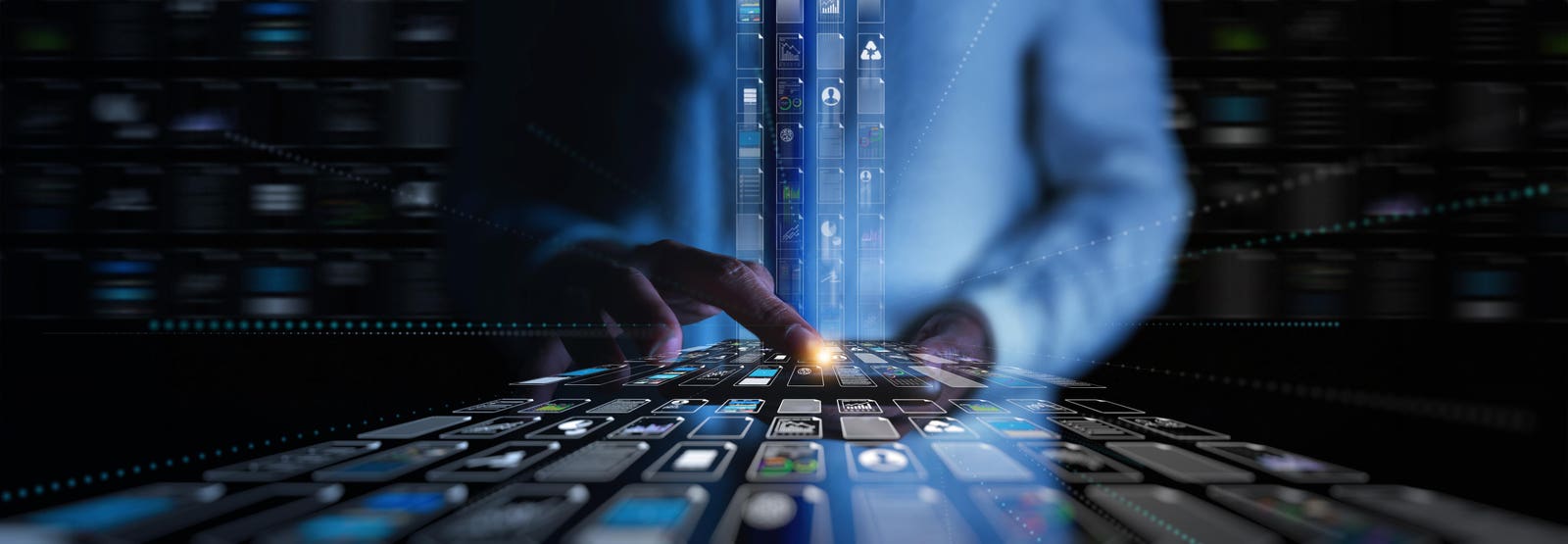
Trust in the data behind artificial intelligence and other data-driven initiatives is going downhill – fast. A recent survey finds fewer than half of business leaders say they have the data they need to pursue cutting-edge strategies – and their numbers have declined somewhat dramatically over the past two years.
The Current State of Data Trust
That’s the word from a recent Salesforce survey of 552 business leaders, which finds trust in the data that underpins data-driven decisions is falling. For example, only 40% trust the reliability of their companies’ data – down from 54% in a similar survey conducted in 2023. Likewise, 41% say their data is relevant – down from 50% two years ago. And, stunningly, a mere 36% believe their data is accurate – down from 49% just two years ago.
Challenges in Data Analysis
While 63% of leaders say finding, analyzing, and interpreting data on their own is key to their jobs, 54% of them aren’t fully confident in their ability to do this. This lack of confidence is a significant issue, as executives understand that subpar data collection, cleansing, and curation processes can lead to unreliable decision-making.
The Impact of Poor Data on AI Initiatives
Industry observers agree that data poses a problematic issue for AI initiatives of all kinds. Executives are aware that feeding low-quality data into AI models can result in poor decision-making. When it comes to automated processes, the stakes get even higher. “You better have data to back it up, and that’s a problem” said industry analyst Andy Thurai. “Executives are afraid their data is not complete, not wholesome, not timely, stale, not reliable, or not accurate.”
The Role of Synthetic Data
Complicating the situation is the fact that many enterprises now use synthetic data to train their AI models when not enough data is available, or to maintain security. The problem is that “executives’ confidence that the models are trained on real-world data is not there,” Thurai added. This lack of confidence can lead to hesitation in adopting AI solutions.
The Need for a New Approach
Part of the issue may also be the fact that applications designed for the 2020s are relying on technology designed in the 1990s. Enterprises “often struggle with a data infrastructure built over many years that utilizes many different technologies, and was built without a clear plan or direction,” said Dwaine Plauche, senior manager of product marketing for Aspen Technology. What is required is a shift in thinking for the handling and positioning of enterprise data.
Rethinking Data Infrastructure
Plauche advised that enterprises should rethink their data infrastructure as an internal customer service. “The goal should be providing internal customers or projects with needed data – a service-oriented mindset that is aligned with the organization’s strategy.” This approach can help to improve data quality and increase confidence in AI decision-making.
The Role of AI in Data Management
Of course, AI itself can help manage and overcome issues with data. “An adage among veteran data scientists is that good modeling is 80% data preparation and only 20% modeling and analysis,” said Richard Sonnenblick, chief data scientist at Planview. AI can help to identify and filter errors in data, lowering the chances of leading a team to false conclusions.
Using AI to Solve Business Problems
Sonnenblick advised that leaders should start with the business problem and work backwards. “AI for AI’s sake is unlikely to achieve useful results. Leaders should identify the desired outcome of a particular task that AI will support, such as decreased time to completion or a higher success rate. AI excels at identifying patterns in data that are too complex to be quickly recognized by humans, meaning it can provide powerful insights for reassigning tasks or reallocating resources to maximize margins or minimize downtime.”
Conclusion
In conclusion, trust in data is declining, and this is having a significant impact on AI initiatives. To overcome this issue, enterprises need to rethink their data infrastructure and approach to data management. By using AI to manage and overcome issues with data, and by starting with the business problem and working backwards, leaders can increase confidence in AI decision-making and achieve better outcomes.
FAQs
- Q: What is the current state of trust in data?
A: Trust in data is declining, with only 40% of business leaders trusting the reliability of their companies’ data. - Q: What is the impact of poor data on AI initiatives?
A: Poor data can lead to unreliable decision-making and a lack of confidence in AI models. - Q: How can AI help with data management?
A: AI can help to identify and filter errors in data, and provide powerful insights for reassigning tasks or reallocating resources. - Q: What approach should leaders take when using AI to solve business problems?
A: Leaders should start with the business problem and work backwards, identifying the desired outcome of a particular task that AI will support.
-
Career Advice5 months ago
Interview with Dr. Kristy K. Taylor, WORxK Global News Magazine Founder
-
Diversity and Inclusion (DEIA)5 months ago
Sarah Herrlinger Talks AirPods Pro Hearing Aid
-
Career Advice5 months ago
NetWork Your Way to Success: Top Tips for Maximizing Your Professional Network
-
Changemaker Interviews4 months ago
Unlocking Human Potential: Kim Groshek’s Journey to Transforming Leadership and Stress Resilience
-
Diversity and Inclusion (DEIA)5 months ago
The Power of Belonging: Why Feeling Accepted Matters in the Workplace
-
Global Trends and Politics5 months ago
Health-care stocks fall after Warren PBM bill, Brian Thompson shooting
-
Global Trends and Politics5 months ago
Unionization Goes Mainstream: How the Changing Workforce is Driving Demand for Collective Bargaining
-
Training and Development5 months ago
Level Up: How Upskilling Can Help You Stay Ahead of the Curve in a Rapidly Changing Industry