Innovation and Technology
Analytics and Reporting
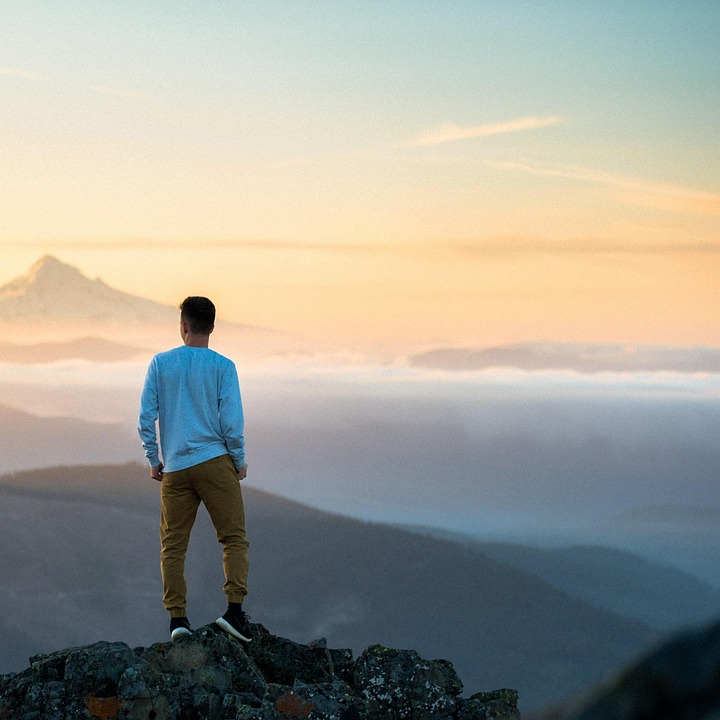
In today’s data-driven world, organizations rely on analytics and reporting to make informed decisions and drive business growth. With the help of software and platforms for Diversity, Equity, Inclusion, and Accessibility (DEIA), companies can now gain a deeper understanding of their operations and make data-driven decisions to improve their bottom line. From tracking website traffic to analyzing customer behavior, analytics and reporting provide valuable insights that can help businesses stay ahead of the competition.
What is Analytics and Reporting?
Analytics and reporting refer to the process of collecting, analyzing, and interpreting data to extract meaningful insights and patterns. This process helps organizations understand their strengths, weaknesses, opportunities, and threats, and make data-driven decisions to drive business growth. Analytics and reporting involve using various tools and techniques, such as data visualization, statistical modeling, and machine learning, to extract insights from large datasets.
Types of Analytics
There are several types of analytics, including descriptive, diagnostic, predictive, and prescriptive analytics. Descriptive analytics involves analyzing historical data to understand what happened, while diagnostic analytics involves analyzing data to understand why something happened. Predictive analytics involves using statistical models and machine learning algorithms to forecast what may happen in the future, while prescriptive analytics involves using data and analytics to recommend specific actions.
Benefits of Analytics and Reporting
The benefits of analytics and reporting are numerous. Some of the key benefits include improved decision-making, increased efficiency, enhanced customer experience, and better risk management. Analytics and reporting help organizations make informed decisions by providing them with accurate and timely data, which can be used to identify areas of improvement and optimize business processes.
Improved Decision-Making
Analytics and reporting provide organizations with accurate and timely data, which can be used to make informed decisions. By analyzing data, organizations can identify trends, patterns, and correlations, which can help them make better decisions. For example, a company may use analytics to analyze customer behavior and identify areas where they can improve their marketing campaigns.
Increased Efficiency
Analytics and reporting can help organizations streamline their operations and improve efficiency. By analyzing data, organizations can identify areas where they can automate processes, reduce waste, and optimize resources. For example, a company may use analytics to analyze their supply chain and identify areas where they can reduce costs and improve delivery times.
Tools and Techniques for Analytics and Reporting
There are several tools and techniques that organizations can use for analytics and reporting. Some of the most common tools include Google Analytics, Tableau, Power BI, and Excel. These tools provide organizations with the ability to collect, analyze, and visualize data, and extract meaningful insights and patterns.
Data Visualization
Data visualization is a powerful tool for analytics and reporting. It involves using charts, graphs, and other visualizations to communicate complex data insights in a clear and concise manner. Data visualization can help organizations identify trends, patterns, and correlations, and make data-driven decisions.
Machine Learning
Machine learning is a type of artificial intelligence that involves using algorithms and statistical models to analyze data and make predictions. Machine learning can be used for predictive analytics, such as forecasting customer behavior and identifying areas of risk.
Best Practices for Analytics and Reporting
There are several best practices that organizations can follow for analytics and reporting. Some of the key best practices include defining clear goals and objectives, collecting high-quality data, and using the right tools and techniques.
Defining Clear Goals and Objectives
Defining clear goals and objectives is critical for analytics and reporting. Organizations should define what they want to achieve through analytics and reporting, and identify the key performance indicators (KPIs) that they will use to measure success.
Collecting High-Quality Data
Collecting high-quality data is essential for analytics and reporting. Organizations should ensure that their data is accurate, complete, and consistent, and that it is collected in a timely and efficient manner.
Common Challenges in Analytics and Reporting
There are several common challenges that organizations face in analytics and reporting. Some of the key challenges include data quality issues, lack of skilled personnel, and limited resources.
Data Quality Issues
Data quality issues are a common challenge in analytics and reporting. Organizations may face issues with data accuracy, completeness, and consistency, which can affect the quality of their analytics and reporting.
Lack of Skilled Personnel
Lack of skilled personnel is another common challenge in analytics and reporting. Organizations may not have the necessary skills and expertise to collect, analyze, and interpret data, which can affect the quality of their analytics and reporting.
Future of Analytics and Reporting
The future of analytics and reporting is exciting and rapidly evolving. With the increasing use of big data, artificial intelligence, and machine learning, organizations will have access to more data and analytics tools than ever before.
Big Data
Big data refers to the large amounts of structured and unstructured data that organizations collect and analyze. Big data can provide organizations with valuable insights and patterns, which can be used to make data-driven decisions.
Artificial Intelligence
Artificial intelligence (AI) refers to the use of algorithms and statistical models to analyze data and make predictions. AI can be used for predictive analytics, such as forecasting customer behavior and identifying areas of risk.
Conclusion
In conclusion, analytics and reporting are critical components of any organization’s decision-making process. By using software and platforms for DEIA, organizations can gain a deeper understanding of their operations and make data-driven decisions to drive business growth. With the right tools and techniques, organizations can unlock the power of analytics and reporting and achieve their goals.
Frequently Asked Questions (FAQs)
What is analytics and reporting?
Analytics and reporting refer to the process of collecting, analyzing, and interpreting data to extract meaningful insights and patterns.
What are the benefits of analytics and reporting?
The benefits of analytics and reporting include improved decision-making, increased efficiency, enhanced customer experience, and better risk management.
What tools and techniques are used for analytics and reporting?
Some of the most common tools and techniques used for analytics and reporting include Google Analytics, Tableau, Power BI, Excel, data visualization, and machine learning.
What are the best practices for analytics and reporting?
Some of the key best practices for analytics and reporting include defining clear goals and objectives, collecting high-quality data, and using the right tools and techniques.
What are the common challenges in analytics and reporting?
Some of the common challenges in analytics and reporting include data quality issues, lack of skilled personnel, and limited resources.
Innovation and Technology
The Most Important Job In The AI Revolution
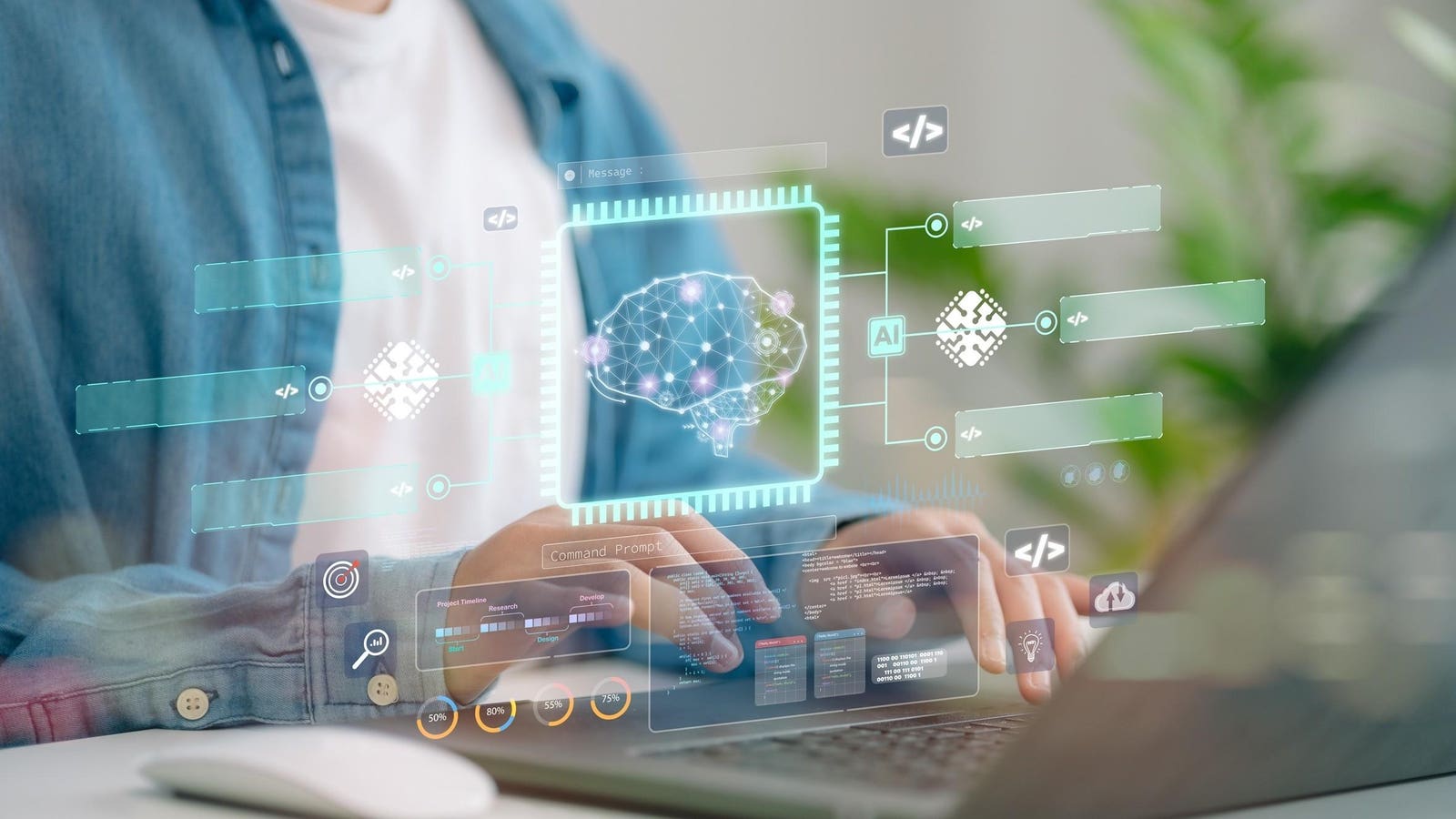
Introduction to the AI Analyst Role
The next wave of business transformation isn’t just about having AI — it’s about having people who can make AI truly understand your business. While headlines focus on engineers building large language models and sophisticated AI agents, a quiet revolution is brewing in the analytics departments of forward-thinking companies: the emergence of the AI analyst.
My recent conversation with Andy MacMillan, CEO of leading enterprise analytics platform Alteryx, highlighted this critical yet underappreciated role in the AI revolution. As MacMillan explains, the gap between raw business data and AI-ready information requires human expertise that combines technical knowledge with deep business acumen.
"I think there’s gonna be a whole set of new roles that emerge because of AI," MacMillan told me. "I think we talk a lot about the technology roles today. People that are building the large language models, people that are building the AI agents. But I think what’s gonna also be needed is the business expertise."
The AI-Data Disconnect
Most organizations have spent decades organizing their data around business applications. Your CRM data is structured to support your CRM software. Your ERP data is designed for ERP functions. But AI needs something different.
"What is essentially the world’s largest data prep and data transformation project is about to start," MacMillan explained. "Companies rethinking what they need to use this data for."
This mismatch creates a fundamental problem. You can’t simply point an AI system at your existing business data and expect it to understand your business processes, company policies, or industry specifics. MacMillan illustrated this with a practical example of sales commissions:
"If you go ask a sales leader, what’s the commission plan? They’re gonna say which team are they on? What region are they in? What does their comp plan look like? What spiffs and programs are we running?" he explained. "You might have to go get their commission plan out of your commission platform. You might have to get their base salary out of your HR database. ‘Cause usually, their commission is a percent of their salary."
What Exactly Is An AI Analyst?
The AI analyst bridges the gap between business knowledge and AI capability. These professionals understand both business operations and how to prepare data to make AI truly valuable.
According to MacMillan, the perfect candidate is "really that mix of data understanding, but business acumen. It’s not just somebody who can do really interesting computer science or data science. You’re gonna have to understand what really makes the business tick."
These analysts take their knowledge of how business processes work and translate that into data workflows that AI can use. They understand which questions need asking and what context is required to make answers meaningful.
"I think for the next decade, there’s gonna be a huge opportunity for everybody that knows how to work with these data sets and understand the business," MacMillan predicted. "These are business users, not simply data scientists. These are people that understand how the data makes the business work."
The AI Data Clearinghouse
Beyond preparing data technically, organizations face governance challenges in determining what information should be accessible to AI systems.
"We have a top-down mandate to use more AI and a top-down mandate that none of our data, our IP, any of our information can be put into any AI tool," MacMillan said, describing the contradictory directions he’s heard from multiple customers.
This is where the concept of an "AI data clearinghouse" becomes essential — a systematic approach to reviewing, approving, and managing what data feeds into AI systems.
"You can have a process where you go back to the clearinghouse," MacMillan explained. "You go into a data workflow like one you might build with Alteryx, and you say, okay, in this workflow, this data flow, I’m gonna pull out this information, and I’m gonna republish this workflow. I’m gonna get sign-off again."
This formal structure helps organizations balance innovation with compliance and security concerns. It creates an auditable, manageable system for making business information available to AI tools.
From Dashboards To AI-Generated Insights
The AI transformation is also changing how we interact with analytics. Instead of static dashboards requiring human interpretation, AI can deliver narrative reports highlighting the most important insights.
"Imagine instead of visualization being I go to a dashboard, I go to my big company dashboard, and it’s all color-coded, and I’m clicking around trying to figure out what’s going on. What if, instead, you were just getting a report that was telling you what’s going on?" MacMillan suggested.
Alteryx is investing in this capability with what they call "Magic Reports" — AI-generated analyses that explain what’s happening rather than just displaying data.
"My example earlier about sales being down in the Southwest is my made-up example, right? What if I was getting a report, my sales report every week, and my sales report wasn’t just a set of numbers, but it was an analysis," MacMillan elaborated. "Hey, Andy, here’s your report. Here’s how things are going. By the way, sales are down in the Southwest; you’ll notice this."
The Future Of Business Analytics
The transformation of enterprise data for AI use represents a fundamental shift in how organizations think about their information assets. While current AI implementations often focus on creative tasks using unstructured data, the real business value will come from applying AI to structured business data.
"Today, the most powerful use cases around AI tend to be, I’m using creative works to create other creative works," MacMillan noted. "That’s different than asking it, what’s going on in the Southwest region and having it understand how to analyze your business."
This transition raises strategic questions about what systems and capabilities organizations should own versus purchase. Will companies rely on AI agents from their existing software vendors, build proprietary systems, or create hybrid approaches?
"I think the next five years in tech are gonna be more interesting than any five years, maybe since the .com boom," MacMillan predicted. "As people really rethink what’s possible."
The People Behind The AI Revolution
While many worry about AI replacing jobs, MacMillan sees tremendous opportunity, particularly for those in analytics who embrace these changes.
"For some folks, that’s a pretty big concern. But I think if you’re in the data and analytics space, there’s gonna be a big need for a lot of knowledge on how businesses operate," he said.
The AI analyst role represents not just a new job title but a reflection of how AI is reshaping business. The most successful implementations won’t be those with the most sophisticated algorithms but those that best combine technological capabilities with human business expertise.
As organizations navigate this transition, those who recognize the importance of the human element in making AI truly business-ready will gain significant advantages in turning artificial intelligence into genuine business intelligence.
Conclusion
In conclusion, the emergence of the AI analyst role is a critical development in the AI revolution. As organizations strive to make their data AI-ready, they will need professionals who can bridge the gap between business knowledge and AI capability. The AI analyst role represents a new opportunity for those in the data and analytics space, and those who embrace this change will be well-positioned to succeed in the future of business analytics.
FAQs
Q: What is an AI analyst?
A: An AI analyst is a professional who bridges the gap between business knowledge and AI capability, understanding both business operations and how to prepare data to make AI truly valuable.
Q: What is the AI-data disconnect?
A: The AI-data disconnect refers to the mismatch between the way organizations have traditionally structured their data and the needs of AI systems.
Q: What is an AI data clearinghouse?
A: An AI data clearinghouse is a systematic approach to reviewing, approving, and managing what data feeds into AI systems, helping organizations balance innovation with compliance and security concerns.
Q: How is the AI transformation changing business analytics?
A: The AI transformation is changing how we interact with analytics, moving from static dashboards to AI-generated insights and narrative reports.
Q: What is the future of business analytics?
A: The future of business analytics will involve applying AI to structured business data, representing a fundamental shift in how organizations think about their information assets.
Innovation and Technology
The Data-Driven Organization: How Technology is Enabling More Effective Decision-Making and Innovation
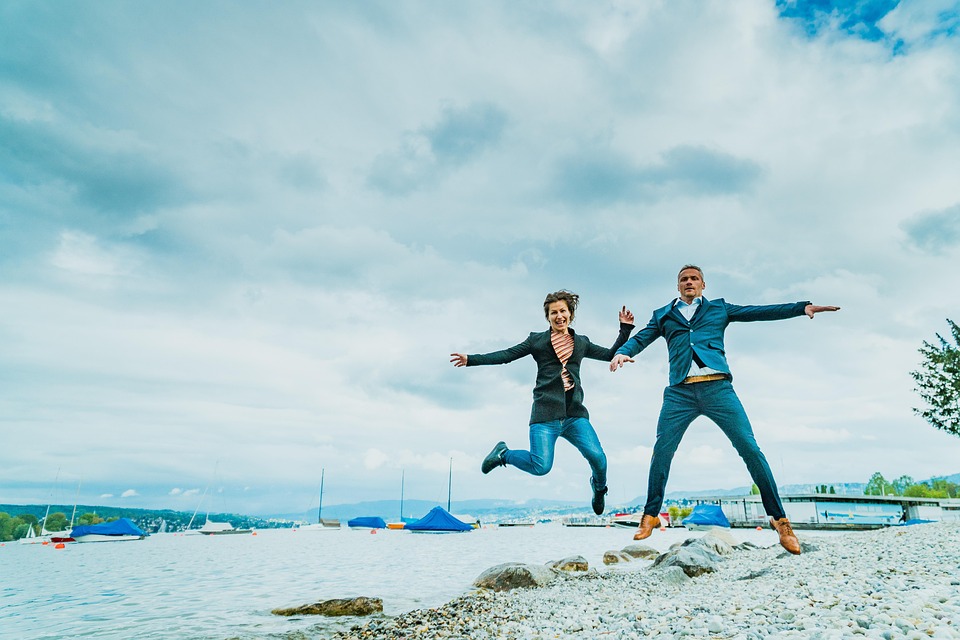
With the increasing adoption of tech-enabled leadership strategies, organizations are now able to make more informed decisions and drive innovation. By leveraging data analytics, artificial intelligence, and other digital technologies, companies can unlock new insights and opportunities for growth. In this article, we’ll explore the ways in which technology is transforming the modern organization and enabling more effective decision-making and innovation.
Embracing a Data-Driven Culture
To become a data-driven organization, companies must first adopt a culture that values data and analytics. This requires a fundamental shift in mindset, from relying on intuition and experience to making decisions based on evidence and data. By doing so, organizations can reduce the risk of bias and errors, and make more informed decisions that drive business outcomes.
Key Characteristics of a Data-Driven Culture
A data-driven culture is characterized by several key elements, including a strong focus on data quality, a culture of experimentation, and a willingness to challenge assumptions. It also requires a high degree of collaboration and communication among teams, as well as a commitment to ongoing learning and development. By fostering these characteristics, organizations can create an environment that is conducive to data-driven decision-making and innovation.
Leveraging Data Analytics and AI
Data analytics and artificial intelligence (AI) are two of the most powerful technologies driving the data-driven organization. By applying advanced analytics and AI techniques to large datasets, companies can unlock new insights and patterns that would be impossible to detect through manual analysis.
Applications of Data Analytics and AI
The applications of data analytics and AI are numerous and varied, from predictive maintenance and quality control to customer segmentation and personalized marketing. By leveraging these technologies, organizations can optimize their operations, improve customer engagement, and drive revenue growth. Additionally, data analytics and AI can be used to identify new business opportunities and inform strategic decisions.
Enabling Innovation through Digital Transformation
Digital transformation is a critical component of the data-driven organization, enabling companies to innovate and stay ahead of the competition. By leveraging digital technologies such as cloud computing, blockchain, and the Internet of Things (IoT), organizations can create new products, services, and business models that drive growth and revenue.
Key Elements of Digital Transformation
Digital transformation requires several key elements, including a clear vision and strategy, a strong focus on customer experience, and a commitment to ongoing innovation and experimentation. It also requires a high degree of agility and adaptability, as well as a willingness to take calculated risks and challenge traditional ways of working. By embracing these elements, organizations can drive successful digital transformation and stay ahead of the competition.
Building a Tech-Enabled Leadership Team
To drive the data-driven organization, companies need a leadership team that is tech-enabled and data-savvy. This requires a deep understanding of digital technologies and their applications, as well as the ability to communicate complex technical concepts to non-technical stakeholders.
Key Characteristics of Tech-Enabled Leaders
Tech-enabled leaders are characterized by several key elements, including a strong focus on innovation and experimentation, a willingness to challenge traditional ways of working, and a commitment to ongoing learning and development. They are also adept at building and managing high-performing teams, and are able to communicate complex technical concepts to non-technical stakeholders. By fostering these characteristics, organizations can build a leadership team that is equipped to drive the data-driven organization.
Measuring Success in the Data-Driven Organization
To measure success in the data-driven organization, companies need to establish clear metrics and benchmarks that align with their business objectives. This may include metrics such as revenue growth, customer engagement, and return on investment (ROI), as well as metrics that measure the effectiveness of data analytics and AI.
Key Performance Indicators (KPIs)
Key performance indicators (KPIs) are a critical component of measuring success in the data-driven organization. By establishing clear KPIs that align with business objectives, organizations can track progress and make adjustments to their strategy as needed. This may include KPIs such as data quality, analytics adoption, and AI-driven insights, as well as KPIs that measure the effectiveness of digital transformation.
Overcoming Challenges and Barriers
Despite the many benefits of the data-driven organization, there are several challenges and barriers that companies may face. These may include cultural and organizational barriers, such as resistance to change and lack of data literacy, as well as technical barriers, such as data quality and integration.
Strategies for Overcoming Challenges
To overcome these challenges, organizations can employ several strategies, including establishing a clear vision and strategy, building a strong team, and fostering a culture of innovation and experimentation. They can also leverage external partnerships and collaborations to drive innovation and stay ahead of the competition.
Conclusion
In conclusion, the data-driven organization is a powerful model for driving innovation and growth in the digital age. By leveraging data analytics, AI, and digital transformation, companies can unlock new insights and opportunities for growth, and make more informed decisions that drive business outcomes. To achieve success, organizations must adopt a culture that values data and analytics, build a tech-enabled leadership team, and establish clear metrics and benchmarks for measuring success. By doing so, they can overcome the challenges and barriers that stand in their way, and achieve their full potential in the data-driven economy.
Frequently Asked Questions (FAQs)
What is a data-driven organization?
A data-driven organization is a company that uses data and analytics to inform its decision-making and drive business outcomes. This requires a culture that values data and analytics, as well as the use of digital technologies such as data analytics and AI.
How can I build a data-driven culture in my organization?
To build a data-driven culture, you need to establish a clear vision and strategy, build a strong team, and foster a culture of innovation and experimentation. You also need to provide ongoing training and development opportunities to help employees build their data literacy and analytics skills.
What are the benefits of using data analytics and AI in my organization?
The benefits of using data analytics and AI include improved decision-making, increased efficiency, and enhanced customer engagement. Data analytics and AI can also be used to drive innovation and stay ahead of the competition.
How can I measure the success of my data-driven organization?
To measure the success of your data-driven organization, you need to establish clear metrics and benchmarks that align with your business objectives. This may include metrics such as revenue growth, customer engagement, and return on investment (ROI), as well as metrics that measure the effectiveness of data analytics and AI.
What are the challenges and barriers to becoming a data-driven organization?
The challenges and barriers to becoming a data-driven organization include cultural and organizational barriers, such as resistance to change and lack of data literacy, as well as technical barriers, such as data quality and integration. To overcome these challenges, you need to establish a clear vision and strategy, build a strong team, and foster a culture of innovation and experimentation.
Innovation and Technology
Longevity Revolution
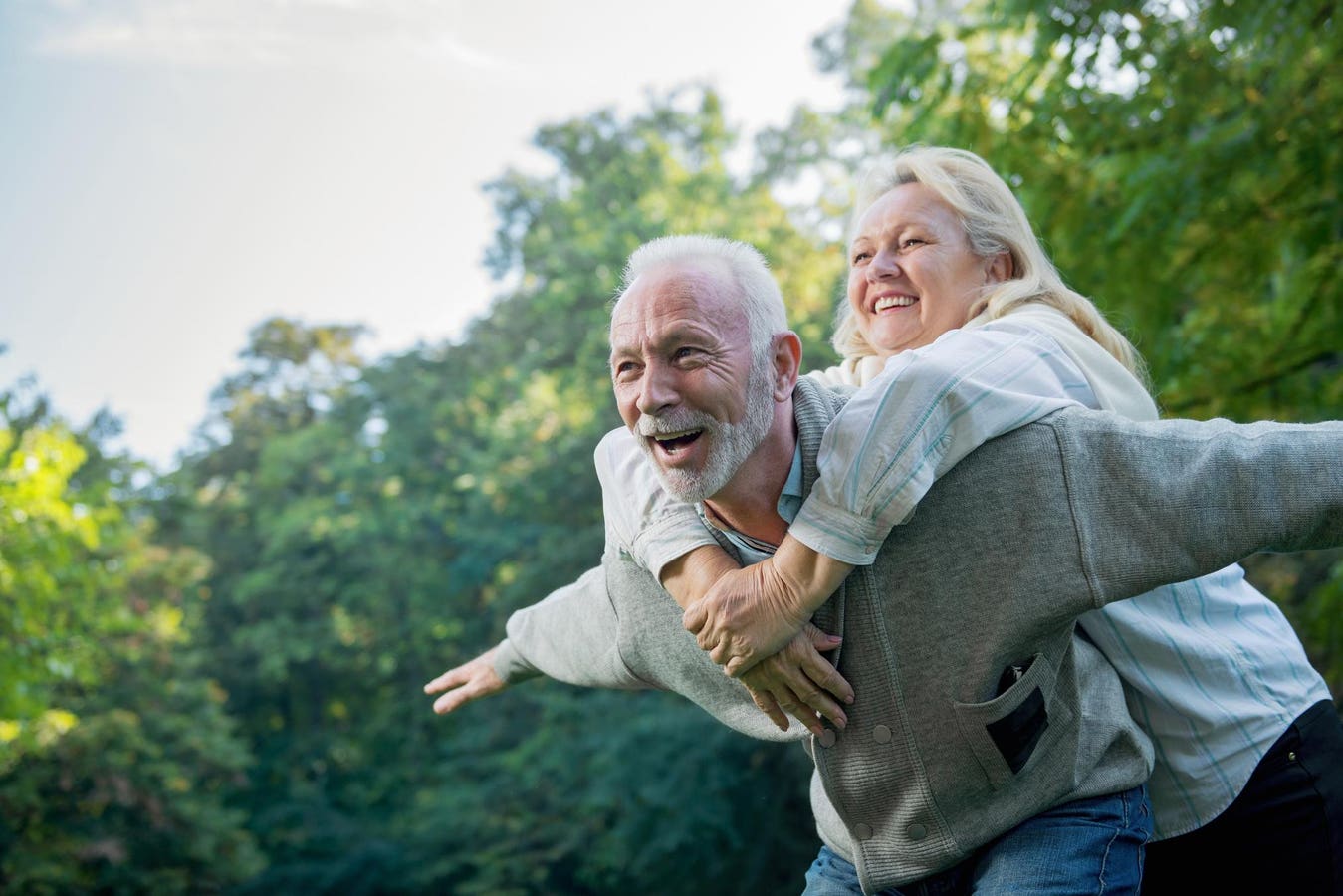
Introduction to Longevity
Aging isn’t what it used to be. For centuries, we’ve accepted it as an inevitable decline—a slow march toward frailty and disease. But what if we’ve been thinking about aging all wrong? Across science labs, venture capital firms, and boardrooms, a growing number of experts see longevity not as a passive process but as an active challenge—one that can be managed, delayed, and even reversed. The longevity industry is no longer science fiction. It’s here, and it’s poised to reshape everything from healthcare to retirement, workforce dynamics, and even the way we structure our economies.
Why Now? The Demographic and Economic Shift
By 2050, over 2.1 billion people—more than 20% of the global population—will be over 60. That’s a seismic shift with enormous implications. Aging populations bring rising healthcare costs, labor shortages in elder care, and mounting pressure on pension systems. But they also present an opportunity: the chance to extend not just life span but health span—the number of years we live in good health, free from chronic disease. Governments and policymakers are taking notice. Countries like Japan, facing some of the world’s fastest-aging populations, are actively rethinking how they approach health, work, and social support structures. The challenge is clear: ensuring that these extra years are filled with vitality and independence rather than illness and dependency.
The Science That’s Changing Everything
For decades, we assumed aging was simply a natural, unstoppable process. But recent breakthroughs suggest otherwise. Researchers now believe biological age—how old our cells and tissues functionally behave—can be decoupled from chronological age. Take epigenetic reprogramming, a field pioneered by Harvard scientists, which aims to restore youthful function to aging cells. Or senolytics, a new class of drugs designed to eliminate "zombie cells" that contribute to inflammation and age-related diseases. The momentum is real: from 2021 to 2023, over $18 billion flowed into longevity-focused research and startups. AI is accelerating these breakthroughs, dramatically speeding up drug discovery and diagnostics. With machine learning models analyzing vast datasets of genetic, metabolic, and lifestyle factors, biotech firms are identifying promising anti-aging compounds faster than ever before.
Big Money is Betting on Longevity
Where there’s innovation, there’s investment. And longevity is attracting some of the biggest names in business and tech. Jeff Bezos has backed Altos Labs, a startup exploring cellular rejuvenation. Google’s Calico Labs is diving deep into age-related diseases, leveraging AI and molecular biology. Even consumer brands are pivoting. L’Oréal is partnering with biotech firms to develop skin-care products that target biological aging. Nestlé is exploring longevity-focused nutrition. Abbott’s Lingo wearable device is providing real-time metabolic insights, empowering consumers to take control of their health spans. Venture capital is pouring into the space, signaling that longevity isn’t just an abstract concept—it’s the next trillion-dollar industry, set to transform healthcare, wellness, and economic structures alike.
The Commercialization of Longevity
Longevity is no longer confined to the realm of biotech startups and research labs. It’s rapidly moving into mainstream consumer markets, with three major industries leading the charge:
- Nutrition: Companies like Elysium Health and Tally Health are offering science-backed supplements and personalized DNA-based longevity plans.
- Wearable technology: Devices like the Oura Ring and Apple Watch are evolving into full-fledged biohacking tools, giving users real-time insights into their biological age and metabolic health.
- Beauty and skincare: The skincare industry is going beyond anti-wrinkle creams, investing in treatments that work at the cellular level to slow skin aging and even regenerate damaged tissue.
What Happens Next?
As longevity becomes mainstream, its ripple effects will extend far beyond healthcare.
- Financial services: Insurance and pension models will have to adapt. Should a 40-year-old with a biological age of 25 pay the same life insurance premiums as someone whose biological age is 60?
- Workforce and retirement: If people remain healthier for longer, retirement ages may shift. Companies might need to rethink workforce policies, reskilling initiatives, and even the traditional career arc.
- Ethical considerations: Will longevity treatments be accessible to all, or will they become the privilege of the ultra-wealthy? With 80% of the world’s aging population expected to be in low- and middle-income countries by 2050, ensuring equitable access to these breakthroughs is critical.
Conclusion
The longevity industry is at an inflection point. We may not be living to 150 just yet, but the quest to redefine aging is already reshaping healthcare, business, and consumer expectations. For businesses and investors, this isn’t just another passing trend. It’s a fundamental reimagining of human potential. Those who recognize this shift and invest early will be at the forefront of a new economic transformation—one where age is no longer a limitation, but a challenge to be overcome.
FAQs
-
What is the longevity industry, and how is it changing?
- The longevity industry refers to the sector focused on extending human lifespan and healthspan through various means, including biotechnology, artificial intelligence, and personalized medicine. It is changing by moving beyond traditional healthcare approaches to incorporate preventive measures, regenerative technologies, and lifestyle interventions.
-
How does biological age differ from chronological age?
- Biological age refers to the functional age of an individual’s cells and tissues, which can be different from their chronological age. Factors such as lifestyle, genetics, and exposure to stress can influence biological age, making it possible for someone to have a biological age that is higher or lower than their chronological age.
-
What role does AI play in the longevity industry?
- AI plays a significant role in the longevity industry by accelerating drug discovery, improving diagnostics, and personalizing treatment plans. Machine learning models can analyze vast datasets to identify patterns and predict outcomes, leading to more effective interventions and therapies.
-
How might the increasing focus on longevity affect workforce dynamics and retirement?
- The increasing focus on longevity could lead to changes in workforce dynamics and retirement, as people remain healthier and more productive for longer. This might result in later retirement ages, the need for reskilling and upskilling, and a reevaluation of traditional career paths and pension systems.
- What are some of the ethical considerations surrounding longevity treatments?
- Ethical considerations include ensuring equitable access to longevity treatments, addressing potential inequalities in access based on socioeconomic status, and considering the societal implications of increased human lifespan, such as impacts on social security systems and workforce structures.
-
Career Advice4 months ago
Interview with Dr. Kristy K. Taylor, WORxK Global News Magazine Founder
-
Diversity and Inclusion (DEIA)4 months ago
Sarah Herrlinger Talks AirPods Pro Hearing Aid
-
Career Advice4 months ago
NetWork Your Way to Success: Top Tips for Maximizing Your Professional Network
-
Changemaker Interviews3 months ago
Unlocking Human Potential: Kim Groshek’s Journey to Transforming Leadership and Stress Resilience
-
Diversity and Inclusion (DEIA)4 months ago
The Power of Belonging: Why Feeling Accepted Matters in the Workplace
-
Global Trends and Politics4 months ago
Health-care stocks fall after Warren PBM bill, Brian Thompson shooting
-
Global Trends and Politics4 months ago
Unionization Goes Mainstream: How the Changing Workforce is Driving Demand for Collective Bargaining
-
Training and Development4 months ago
Level Up: How Upskilling Can Help You Stay Ahead of the Curve in a Rapidly Changing Industry