Innovation and Technology
How AI Is Transforming 70% Of Jobs By 2030
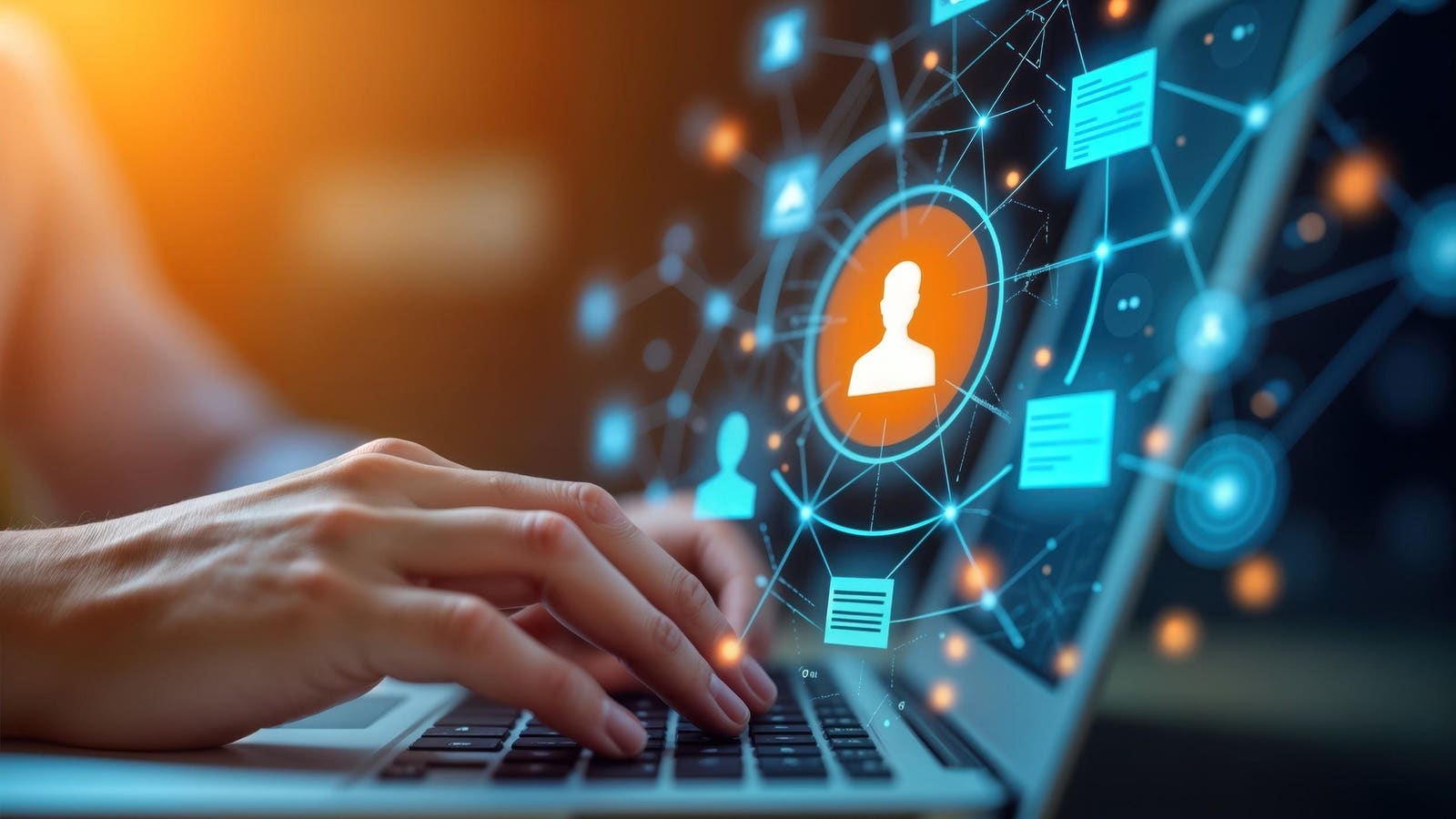
Innovation and Technology
Overcoming Overthinking
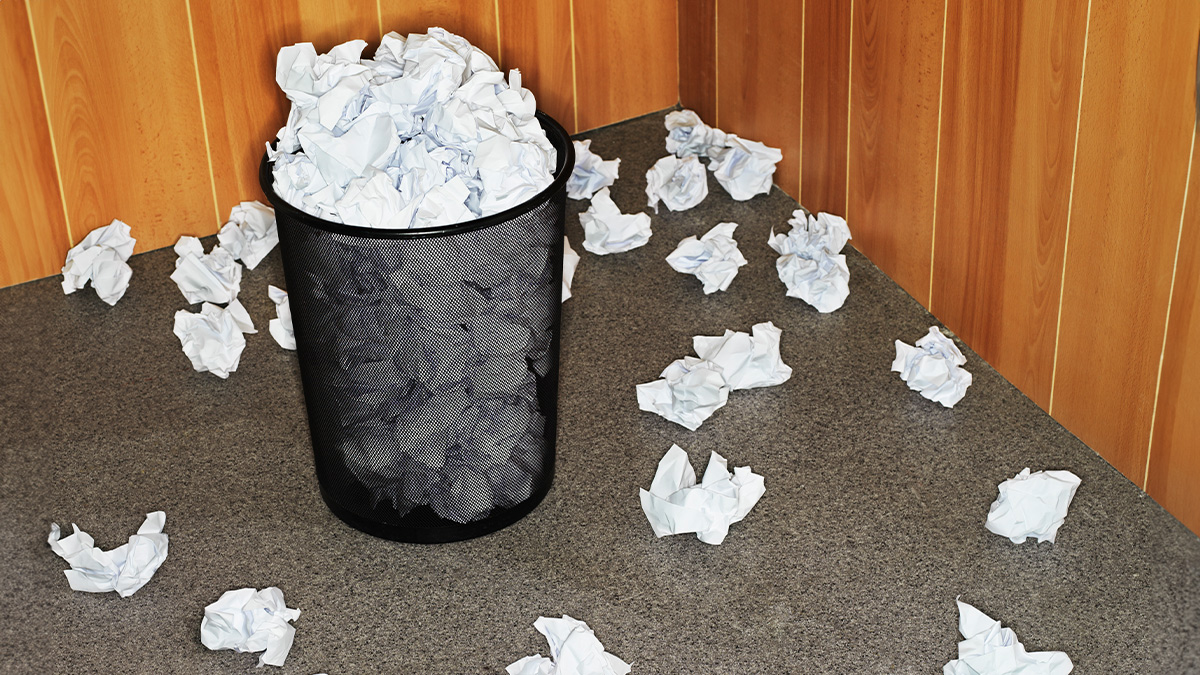
Introduction to Overthinking
Overthinking is a common phenomenon that can affect anyone, regardless of their background or circumstances. It involves excessive thinking about a particular situation, problem, or issue, which can lead to anxiety, stress, and decreased productivity. There are several types of overthinking, and understanding them is essential to overcoming them.
Types of Overthinking
There are three primary types of overthinking: ruminative thinking, catastrophic thinking, and analytical thinking. Each type has distinct characteristics and effects on an individual’s mental and emotional well-being.
Ruminative Thinking
Ruminative thinking involves dwelling on past events or experiences, replaying them in your mind, and rehashing what could have been done differently. This type of thinking can lead to feelings of regret, guilt, and self-blame. Ruminative thinking can be overwhelming and make it challenging to focus on the present moment.
Examples of Ruminative Thinking
Examples of ruminative thinking include:
- Replaying a conversation in your head and thinking about what you should have said
- Dwelling on past mistakes and wondering what could have been done differently
- Reliving memories of past traumas or painful experiences
Catastrophic Thinking
Catastrophic thinking involves imagining the worst-case scenario in any given situation. This type of thinking can lead to anxiety, fear, and a sense of hopelessness. Catastrophic thinking can be debilitating and make it challenging to make decisions or take action.
Examples of Catastrophic Thinking
Examples of catastrophic thinking include:
- Assuming the worst possible outcome in any situation
- Imagining that a minor setback will lead to a major disaster
- Believing that a problem is insurmountable and cannot be solved
Analytical Thinking
Analytical thinking involves overanalyzing information, weighing pros and cons, and considering multiple perspectives. While analytical thinking can be beneficial in certain situations, excessive analysis can lead to indecision, procrastination, and anxiety.
Examples of Analytical Thinking
Examples of analytical thinking include:
- Spending excessive time researching and weighing options
- Considering multiple scenarios and outcomes
- Overthinking the potential consequences of a decision
Overcoming Overthinking
Overcoming overthinking requires self-awareness, strategies, and practice. Here are some tips to help you overcome the different types of overthinking:
Strategies for Overcoming Ruminative Thinking
- Practice mindfulness and focus on the present moment
- Engage in physical activity or exercise to distract yourself from negative thoughts
- Challenge negative thoughts by reframing them in a positive or realistic light
Strategies for Overcoming Catastrophic Thinking
- Challenge negative thoughts by asking yourself if they are based in reality
- Practice relaxation techniques, such as deep breathing or meditation, to calm your mind
- Focus on the present moment and what you can control
Strategies for Overcoming Analytical Thinking
- Set a time limit for decision-making and analysis
- Practice trusting your instincts and making decisions based on your values and goals
- Seek input from others to gain new perspectives and insights
Conclusion
Overthinking can be a significant obstacle to mental and emotional well-being. By understanding the different types of overthinking and implementing strategies to overcome them, you can reduce stress, anxiety, and indecision. Remember that overcoming overthinking takes time and practice, so be patient and compassionate with yourself as you work to develop new thought patterns and habits.
FAQs
Q: What is the difference between overthinking and critical thinking?
A: Overthinking involves excessive thinking that can lead to anxiety and indecision, while critical thinking involves analyzing information to make informed decisions.
Q: How can I stop overthinking at night?
A: Practice relaxation techniques, such as deep breathing or meditation, and avoid screens before bedtime to help calm your mind.
Q: Can overthinking be a sign of a mental health condition?
A: Yes, overthinking can be a symptom of anxiety, depression, or other mental health conditions. If you are concerned about your mental health, consult a mental health professional for guidance and support.
Innovation and Technology
Phone Addiction Test
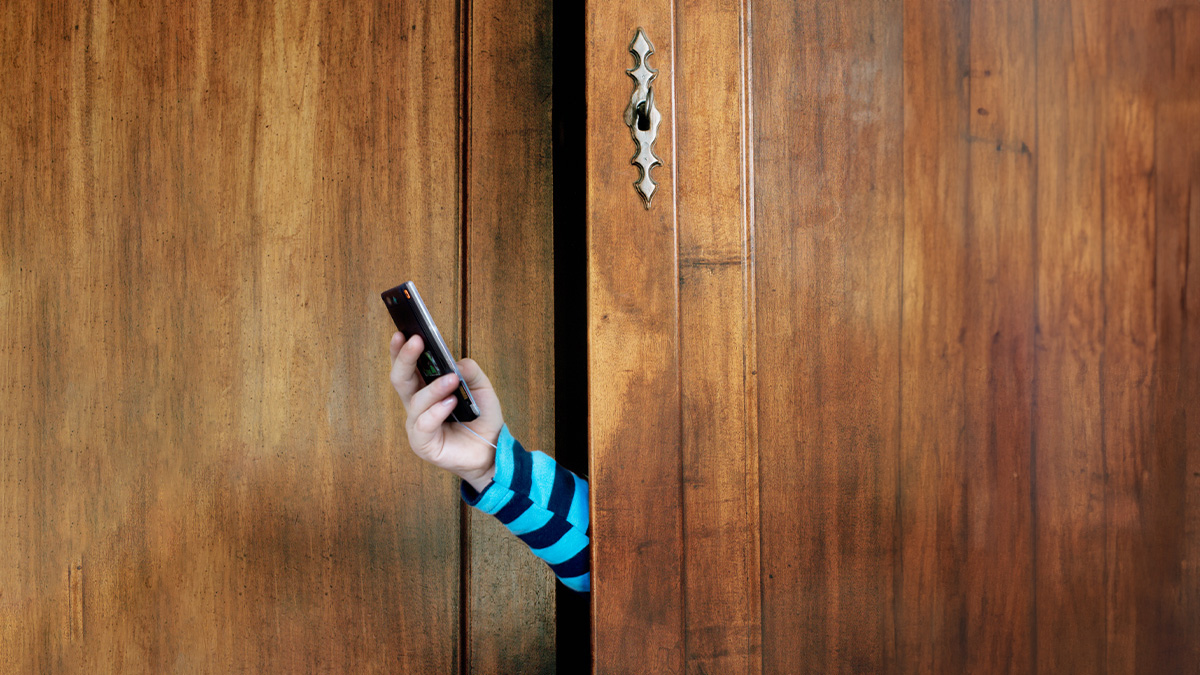
Introduction to the Experiment
During a recent executive program, we conducted a micro-experiment. Participants opted to surrender their mobile phones for one evening and get them back the next morning.
Reflection and Observations
Participants were asked to reflect on their thoughts, emotions, and behaviors during the experiment. The next morning was full of discussion: Some had noticed themselves reaching for their phones mindlessly, coupled with jolts of “panic” when finding it missing; some felt irritable or frustrated about not being able to look things up on demand; some were nervous to wander the city’s streets without their GPS; while others rationalized the reasons they urgently needed their phone or felt extreme fear of missing out.
Outcomes and Insights
At the same time, many felt liberated, noticing more around them and enjoying the freedom of not accessing work emails in the evening. Almost all learned something about themselves.
Conclusion
The experiment provided valuable insights into the participants’ relationship with their mobile phones. It highlighted the emotional attachment people have with their devices and how they can impact daily life. By surrendering their phones, participants were able to identify their mindless habits, experience a range of emotions, and appreciate the freedom that comes with being disconnected. The experiment served as a catalyst for self-reflection, allowing participants to gain a deeper understanding of themselves and their dependence on technology.
FAQs
What was the purpose of the experiment?
The purpose of the experiment was to help participants reflect on their thoughts, emotions, and behaviors in relation to their mobile phone use.
What did participants have to do during the experiment?
Participants had to surrender their mobile phones for one evening and return them the next morning.
What were some common reactions during the experiment?
Common reactions included feeling irritable, frustrated, nervous, or experiencing a fear of missing out. However, many participants also felt liberated and enjoyed the freedom from constant connectivity.
What was the outcome of the experiment?
The experiment helped participants learn something about themselves and their relationship with their mobile phones, promoting self-reflection and a deeper understanding of their dependence on technology.
Innovation and Technology
AI Inference Chip Showdown
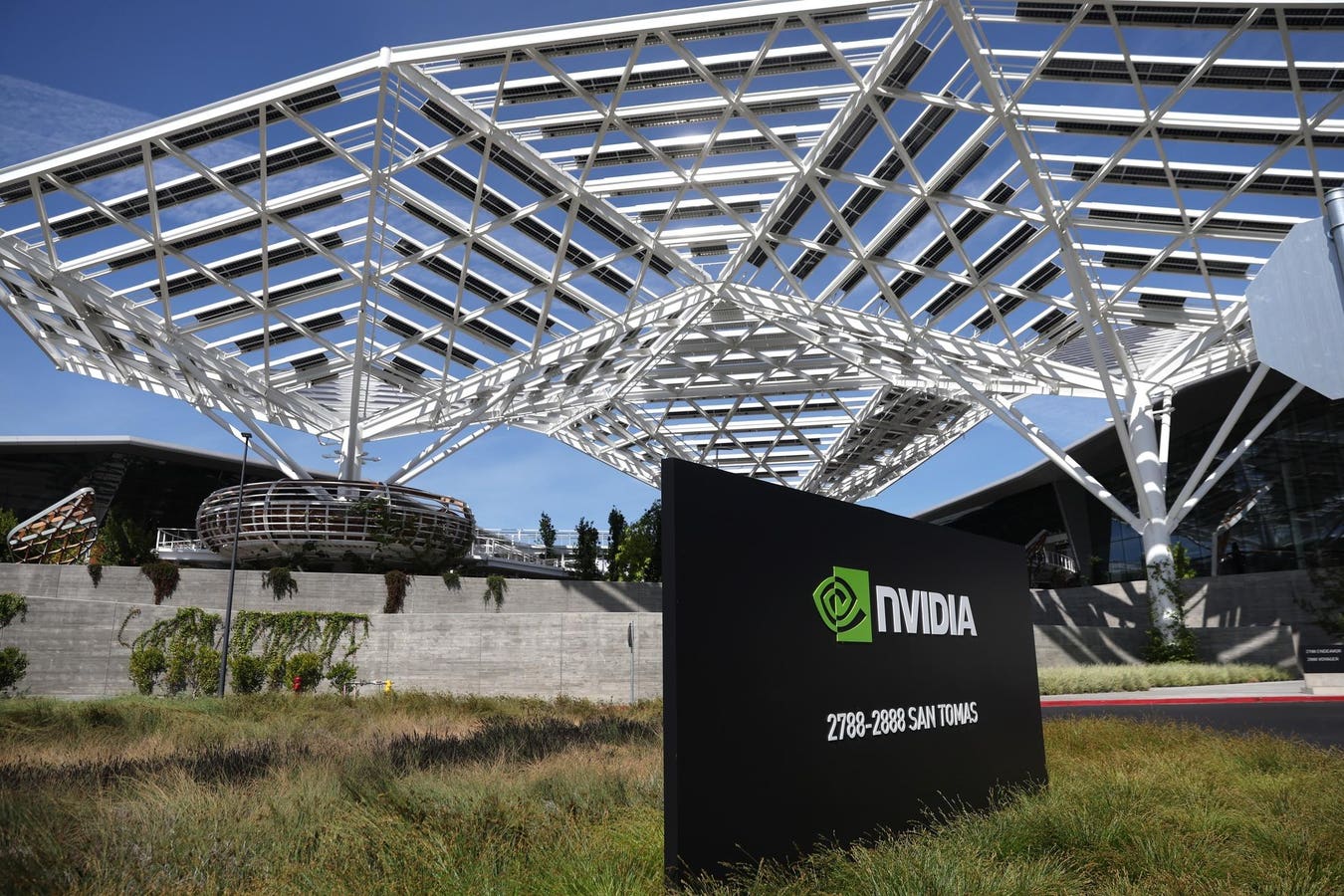
Introduction to AI Inference Processing
Everyone is not just talking about AI inference processing; they are doing it. Analyst firm Gartner released a new report this week forecasting that global generative AI spending will hit $644 billion in 2025, growing 76.4% year-over-year. Meanwhile, MarketsandMarkets projects that the AI inference market is expected to grow from $106.15 billion in 2025 to $254.98 billion by 2030. However, buyers still need to know what AI processor to buy, especially as inference has gone from a simple one-shot run through a model to agentic and reasoning models that can increase computational requirements by some 100-fold.
Performance Continues to Skyrocket
For seven years, the not-for-profit group MLCommons has been helping AI buyers and vendors by publishing peer-reviewed quarterly AI benchmarks. It has just released its Inference 5.0 suite of results, with new chips, servers, and models. Let’s take a look.
The New Benchmarks
New benchmarks were added for the larger Llama 3.1 405B, Llama 2 70B with latency constraints for interactive work, and a new “R-GAT” benchmark for graph models. Only Nvidia ran benchmarks for all the models. A new benchmark was also added for edge inference, the Automotive PointPainting test for 3D object detection. There are now 11 AI benchmarks managed by MLCommons.
The New Chips
AI is built on silicon, and MLCommons received submissions for six new chips this round, including AMD Instinct MI325X (launched last Fall), Intel Xeon 6980P “Granite Rapids” CPU, Google TPU Trillium (TPU v6e) which has become generally available, Nvidia B200 (Blackwell), Nvidia Jetson AGX Thor 128 for AI at the Edge, and perhaps most importantly the Nvidia GB200, the beast that powers the NVL72 rack that has data centers scrambling to power and cool.
The New Results: Nvidia
As usual, Nvidia won all benchmarks; this time, they won by a lot. First, the B200 tripled the performance of the H200 platform, delivering over 59,000 tokens per second on the latency-bounded Llama 2 70B Interactive model. The new Llama 3.2 405B model is 3.4 times faster on Blackwell. Now for the real test: is the NVL72 as fast as Nvidia promised at launch? Yes, it is thirty times faster than the 8-GPU H200 running the new Llama 405B, but it has 9 times more GPUs.
Nvidia Performance
The new Llama 3.1 405B benchmark supports input and output lengths up to 128,000 tokens (compared to only 4,096 tokens for Llama 2 70B). The benchmark tests three distinct tasks: general question-answering, math, and code generation. But when you add Nvidia’s new open-source Dynamo “AI Factory OS” that optimizes AI at the data center level, AI factory throughput can double again running Llama and thirty times faster running DeeSeek.
And, Surprise, AMD Has Rejoined the MLPerf Party!
Welcome back, AMD! The new AMD MI325 did quite well at the select benchmarks AMD ran, competing admirably with the previous generation Hopper GPU. So, for AI practitioners who know what they are doing and don’t need the value of Nvidia software, AMD MI325 can save them a lot of money. AMD also did quite well at the Llama 3.1 405B Serving benchmark (distinct from the interactive 405B benchmark mentioned previously). AMD proudly said that Meta is now using the (older) MI300X as the exclusive inference server for the 405B model.
Conclusion
Nvidia retains the crown of AI King across all AI applications. Although competition is on the horizon, AMD delivers competitive performance only when measured against the previous Nvidia GPU generation. AMD expects that the MI350, due later this year, will close the gap. However, thanks to the GB300, Nvidia will retain the lead at the GPU performance level by then. But the real issue here is that while everyone else is trying to compete at the GPU level, Nvidia keeps raising the bar at the data center level with massive investments in software, solutions, and products to ease AI deployment and lower TCO.
FAQs
- Q: What is the forecast for global generative AI spending in 2025?
A: Global generative AI spending is expected to hit $644 billion in 2025, growing 76.4% year-over-year. - Q: What is the projected growth of the AI inference market from 2025 to 2030?
A: The AI inference market is expected to grow from $106.15 billion in 2025 to $254.98 billion by 2030. - Q: What is the performance of Nvidia’s B200 chip compared to the H200 platform?
A: The B200 chip tripled the performance of the H200 platform, delivering over 59,000 tokens per second on the latency-bounded Llama 2 70B Interactive model. - Q: How does AMD’s MI325 chip perform compared to Nvidia’s Hopper GPU?
A: The AMD MI325 chip competes admirably with the previous generation Hopper GPU, and can save AI practitioners a lot of money if they don’t need Nvidia’s software. - Q: What is the significance of Nvidia’s Dynamo "AI Factory OS"?
A: Nvidia’s Dynamo "AI Factory OS" optimizes AI at the data center level, allowing for doubled throughput and lower TCO.
-
Career Advice4 months ago
Interview with Dr. Kristy K. Taylor, WORxK Global News Magazine Founder
-
Diversity and Inclusion (DEIA)4 months ago
Sarah Herrlinger Talks AirPods Pro Hearing Aid
-
Career Advice4 months ago
NetWork Your Way to Success: Top Tips for Maximizing Your Professional Network
-
Changemaker Interviews3 months ago
Unlocking Human Potential: Kim Groshek’s Journey to Transforming Leadership and Stress Resilience
-
Diversity and Inclusion (DEIA)4 months ago
The Power of Belonging: Why Feeling Accepted Matters in the Workplace
-
Global Trends and Politics4 months ago
Health-care stocks fall after Warren PBM bill, Brian Thompson shooting
-
Global Trends and Politics4 months ago
Unionization Goes Mainstream: How the Changing Workforce is Driving Demand for Collective Bargaining
-
Training and Development4 months ago
Level Up: How Upskilling Can Help You Stay Ahead of the Curve in a Rapidly Changing Industry