Innovation and Technology
Thinking Precedents
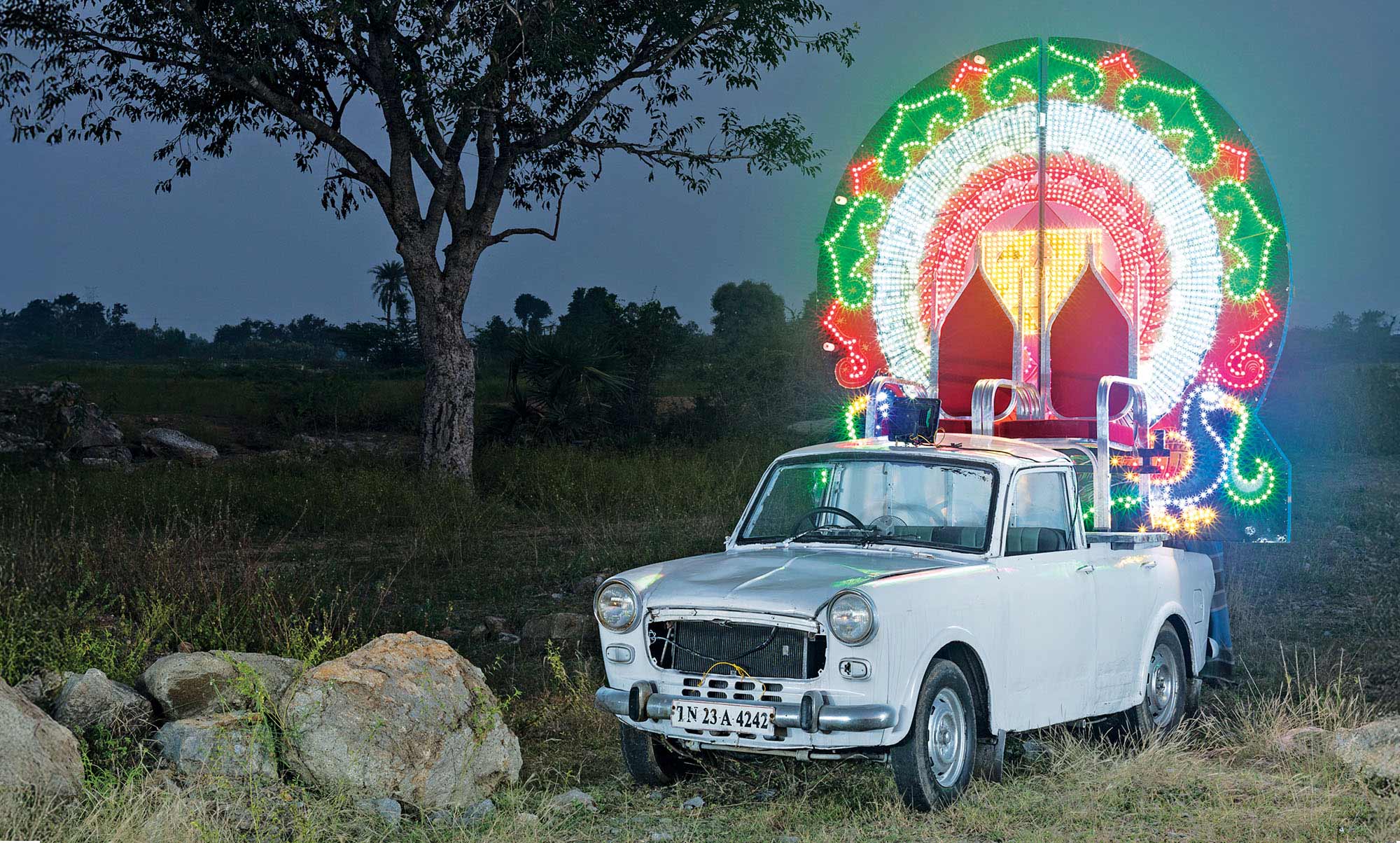
Precedent Thinking: A Framework for Making Better Decisions
What is Precedent Thinking?
Precedent thinking is a decision-making framework that involves considering the precedents, or past decisions, that have been made in similar situations. This approach is based on the idea that history can inform the future, and that by analyzing the outcomes of previous decisions, we can gain valuable insights that can inform our own decision-making.
The Benefits of Precedent Thinking
There are several benefits to using precedent thinking when making decisions. For one, it allows us to learn from the experiences of others, rather than having to start from scratch. This can save time and reduce the risk of making costly mistakes. Additionally, precedent thinking can help us to identify patterns and trends that may not be immediately apparent, and to develop a deeper understanding of the context in which our decisions will be made.
How to Apply Precedent Thinking
So, how can you apply precedent thinking to your own decision-making? Here are a few tips:
* Identify the key factors that led to the desired outcome in the precedent case.
* Analyze the strengths and weaknesses of the precedent decision.
* Consider how the precedent decision might be adapted to fit your own situation.
* Use the insights gained from the precedent decision to inform your own decision-making.
Examples of Precedent Thinking in Action
Precedent thinking can be applied to a wide range of situations, from business and finance to personal relationships and everyday life. Here are a few examples:
* A business leader considering a new marketing strategy might look to past successes or failures for insight on what might work best.
* A person considering a major life change, such as a move to a new city, might look to the experiences of others who have made similar decisions.
* A team leader trying to resolve a complex conflict might look to past resolutions of similar conflicts for guidance.
Conclusion
In conclusion, precedent thinking is a powerful tool for making better decisions. By considering the precedents that have come before us, we can gain valuable insights and avoid costly mistakes. By applying the principles of precedent thinking to our own decision-making, we can make more informed, more effective, and more successful choices.
FAQs
Q: What is the difference between precedent thinking and hindsight bias?
A: While both involve considering past events, precedent thinking is focused on using past decisions to inform future decisions, while hindsight bias is the tendency to overestimate the importance of a particular outcome after it has occurred.
Q: Can precedent thinking be used in non-academic or non-professional settings?
A: Yes, precedent thinking can be applied to any situation where past experiences can inform future decisions.
Q: How do I know if a precedent is relevant to my situation?
A: Consider the key factors that led to the desired outcome in the precedent case. If those factors are similar to your own situation, it may be relevant to consider the precedent.
Innovation and Technology
Sally Ride’s Enduring STEM Legacy
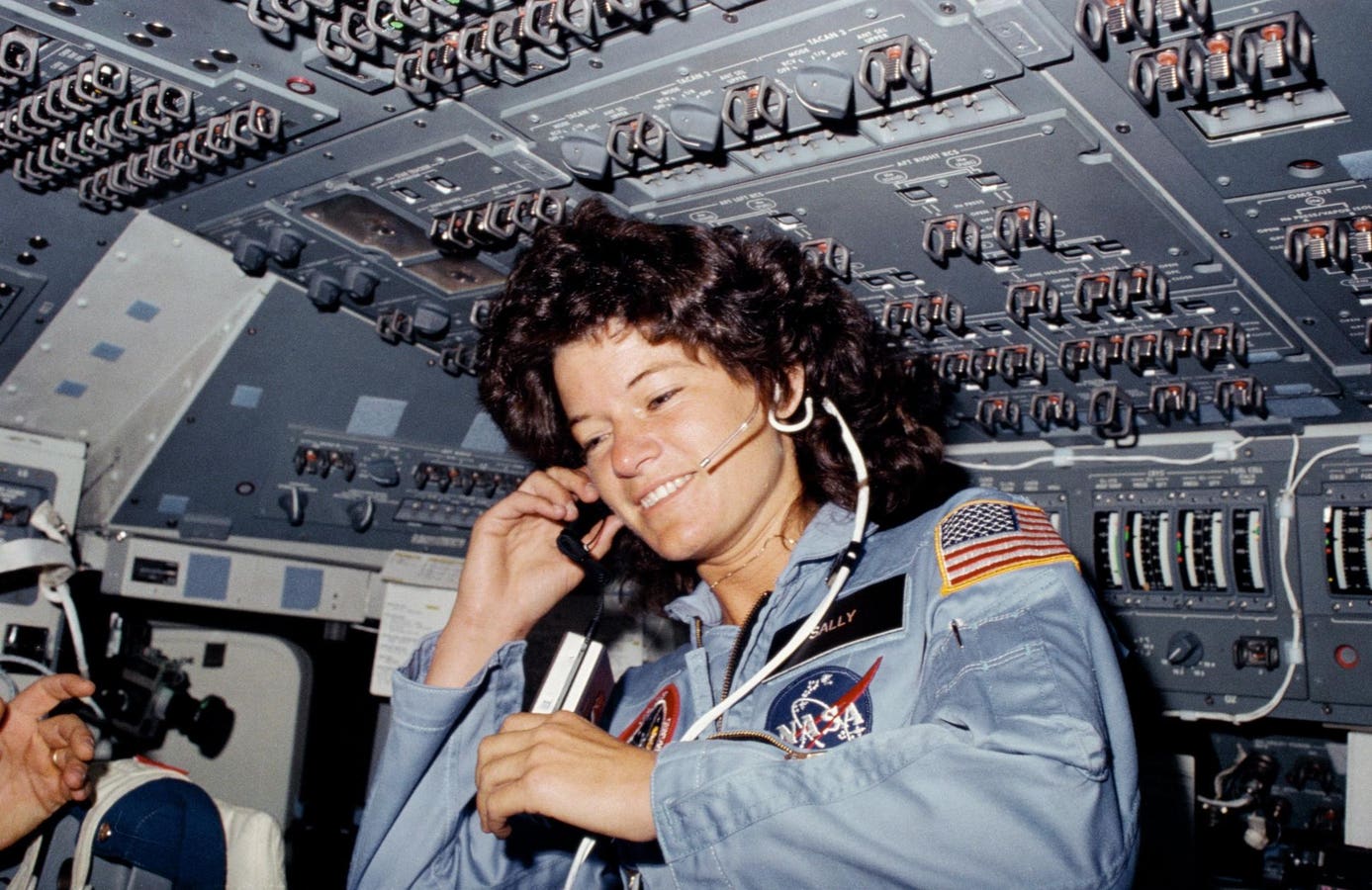
Introduction to Sally Ride
Sally Ride made history on June 18, 1983, when she became the first American woman to launch into space. Her calm precision and unflinching focus made her an icon in STEM—a face of possibility for girls across the country and around the world. But a new documentary from National Geographic, Sally, reveals another dimension of her legacy—one that remained private throughout her lifetime.
The Documentary
Premiering June 16 on National Geographic and streaming on Disney+ and Hulu the next day, Sally tells the story not only of Ride’s groundbreaking achievements as a physicist and astronaut, but of her 27-year partnership with fellow scientist and educator Tam O’Shaughnessy—a relationship Ride never publicly acknowledged while she was alive. Directed by Emmy-winner Cristina Costantini, the film reframes Ride’s legacy, offering a deeper, more personal portrait of a woman who inspired millions while carefully guarding her own truth.
Behind the Documentary
I recently had the privilege of sitting down with O’Shaughnessy and Costantini to better understand the motivation behind the documentary and what they hope audiences will take away from it. What emerged from our conversation was a sense that this film isn’t just about legacy—it’s about visibility, resilience and the complicated cost of breaking barriers in science.
When Representation Requires Erasure
Being first often means carrying the weight of symbolic representation. Ride wasn’t just an astronaut—she was proof that women could thrive in space and science. But with that role came expectations: be competent, but never emotional. Be visible, but only selectively. The unwritten contract Ride operated under demanded technical excellence and personal silence.
The Decision to Share
As Tam told me, the decision to finally share their relationship publicly didn’t begin with a film pitch. It began with grief. “Since about a week before Sally died, I told her I wanted to hold a celebration of her life,” she said. “And then I wondered who I was going to be to the people coming to the celebration who didn’t know we were a couple.” Sally told her, “You decide. Whatever you decide will be the right thing to do.” That moment of quiet affirmation—Ride’s way of granting permission without demanding visibility—was the start of a longer process.
STEM’s Culture of Conformity
Costantini, known for documentaries like Science Fair and Mucho Mucho Amor, wanted Sally to be about more than just history. “We made this film for anyone who’s ever had to hide part of themselves to get where they want to be,” she told me. “In 2025, that experience feels more relevant than ever.” That sense of coded identity—the need to suppress aspects of self to be taken seriously—remains a reality in many corners of science and technology. Despite progress, LGBTQ+ scientists are still underrepresented in leadership roles. Women in STEM continue to navigate environments that value output over authenticity.
Sally’s Enduring Influence in STEM
Even after leaving NASA, Ride continued to shape the STEM world. In 2001, she and O’Shaughnessy founded Sally Ride Science, a nonprofit aimed at promoting STEM education for girls and underrepresented youth. That mission continues today at UC San Diego, where O’Shaughnessy and a dedicated team run programs that connect students with scientists and researchers. It’s not just the curriculum that draws kids in—it’s the name. “Sally’s name is magic,” O’Shaughnessy said. “It’s why the boys and girls sign up, and it’s why parents want their kids to be exposed to these programs.”
A Legacy No Longer Coded
What Sally reveals is a fuller picture of a scientist, a leader and a partner. Someone who not only pushed the boundaries of what women could do in science, but who also made space—quietly, privately—for the people she loved. Ride’s story still resonates because it’s unfinished. It now invites a broader range of people to see themselves in her legacy—not just young girls interested in STEM, but anyone who’s ever felt that they had to choose between their passion and their identity.
Conclusion
For the STEM community, that’s the crux of the problem. The human race has a lot of challenges to address and there are plenty of undiscovered frontiers to tackle. We can’t afford to exclude entire populations of people based on nothing more than fragile egos and narrow-minded bigotry. It’s not enough to recruit diverse talent. We have to build systems where people can bring their full selves to the work. Because progress isn’t just about putting the first woman in space. It’s about making sure she wouldn’t have to hide once she landed.
FAQs
Q: When did Sally Ride become the first American woman to launch into space?
A: Sally Ride made history on June 18, 1983, when she became the first American woman to launch into space.
Q: What is the documentary Sally about?
A: The documentary Sally tells the story of Sally Ride’s groundbreaking achievements as a physicist and astronaut, as well as her 27-year partnership with fellow scientist and educator Tam O’Shaughnessy.
Q: What is the mission of Sally Ride Science?
A: Sally Ride Science is a nonprofit aimed at promoting STEM education for girls and underrepresented youth.
Q: Why is Sally Ride’s legacy still relevant today?
A: Sally Ride’s legacy is still relevant today because it invites a broader range of people to see themselves in her story and because it highlights the importance of creating systems where people can bring their full selves to their work.
Innovation and Technology
Amazon Spending $13 Billion On AI Data Centers In Australia
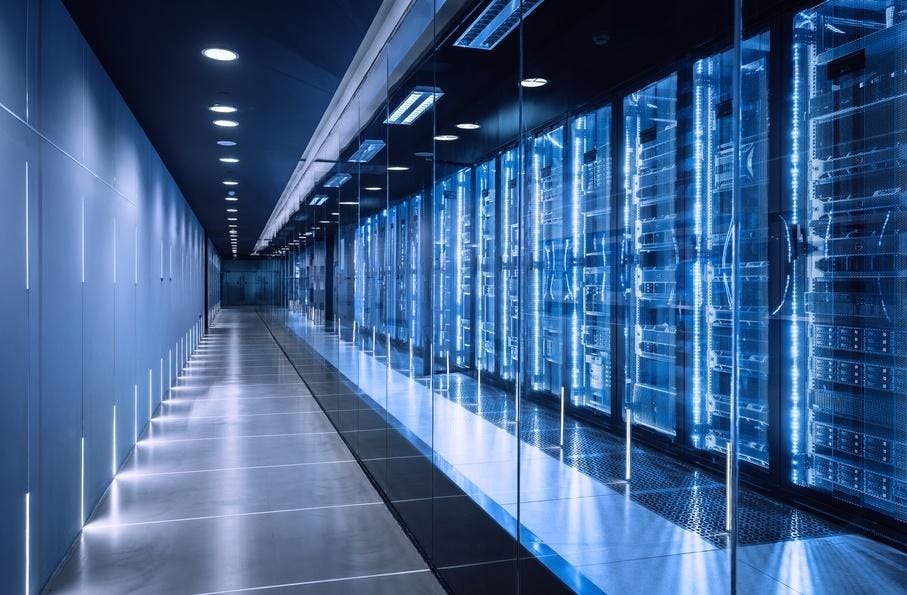
Introduction to Amazon’s Investment in Australia
Amazon will invest A$20 billion ($13 billion) in the five years through 2029 to build data centers in Australia amid growing demand for cloud computing and artificial intelligence applications. The spending—the biggest investment from a global technology provider in Australia—will hasten AI adoption across the country, boost productivity and modernize the IT infrastructure for Australian entities, Amazon said in a statement over the weekend.
Economic Impact and Job Creation
“The investment will generate economic opportunity for Australians, including skilled jobs and infrastructure that can support complex AI and supercomputing applications,” Australian Prime Minister Anthony Albanese said in the statement. Australia’s Department of Industry, Science and Resources estimates AI and automation will contribute up to A$600 billion annually to the nation’s GDP by 2030.
Empowering Innovation at Scale
“AI is a once-in-a-generation transformation, and Amazon is pleased to be empowering all Australians to innovate at scale through this investment,” Matt Garman, CEO of Amazon Web Services, said in the statement. This investment is a significant step towards supporting the growth of AI and cloud computing in Australia.
Supporting Infrastructure for Data Centers
To support the enlarged cloud infrastructure’s power needs, Amazon is investing in three new solar farms in Victoria and Queensland to provide more than 170-megawatts of electricity to the data centers. When completed, Amazon will have 11 solar farms across Australia with a combined capacity of 1.4 million megawatt hours, enough to power about 290,000 Australian homes each year, Amazon said.
History of Amazon’s Investment in Australia
Amazon first invested in Australia in 2012 with the opening of the AWS Asia Pacific (Sydney) Region hub. Since then it has built several new facilities in Melbourne, Perth and Sydney. The e-commerce giant has also trained more than 400,000 people in the country, equipping them with digital skills to support generative AI programs.
Conclusion
Amazon’s significant investment in Australia’s data centers and renewable energy infrastructure underscores the company’s commitment to supporting the country’s digital transformation. This investment is expected to have a profound impact on Australia’s economy, creating new job opportunities and driving innovation in AI and cloud computing.
FAQs
- Q: How much will Amazon invest in Australia’s data centers?
A: Amazon will invest A$20 billion ($13 billion) in the five years through 2029. - Q: What is the expected impact of Amazon’s investment on Australia’s GDP?
A: AI and automation are estimated to contribute up to A$600 billion annually to the nation’s GDP by 2030. - Q: How will Amazon support the power needs of its data centers?
A: Amazon is investing in three new solar farms in Victoria and Queensland to provide more than 170-megawatts of electricity to the data centers. - Q: How many people has Amazon trained in digital skills in Australia?
A: Amazon has trained more than 400,000 people in the country, equipping them with digital skills to support generative AI programs.
Innovation and Technology
Strategic Subtraction
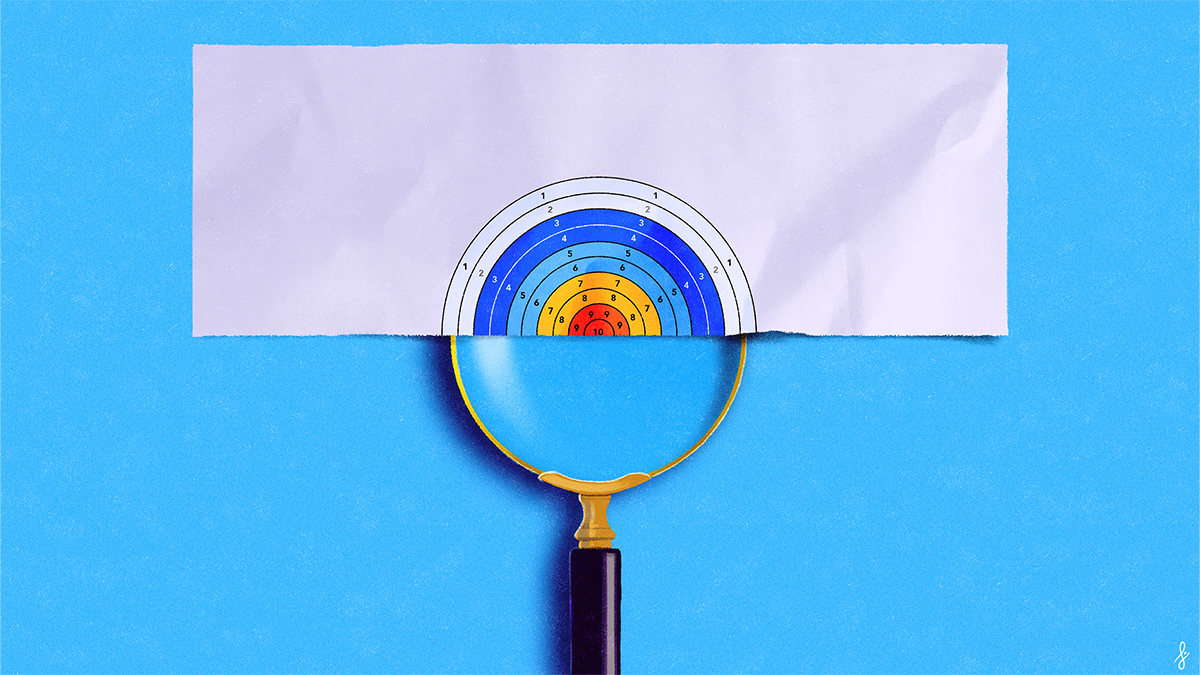
Introduction to Strategic Subtraction
In today’s fast-paced and ever-changing business landscape, companies are constantly seeking ways to stay ahead of the competition and adapt to turbulent times. One strategy that has gained attention in recent years is "strategic subtraction," a concept that involves intentionally removing or simplifying elements of a business to improve its overall performance and resilience.
What is Strategic Subtraction?
Strategic subtraction is a deliberate approach to eliminating non-essential components, processes, or activities that do not contribute significantly to a company’s core objectives. This can include anything from streamlining product lines and simplifying operational processes to reducing the number of employees or closing underperforming locations. The goal of strategic subtraction is to create a more agile, focused, and efficient organization that is better equipped to navigate uncertainty and capitalize on new opportunities.
Benefits of Strategic Subtraction
The benefits of strategic subtraction are numerous and can have a significant impact on a company’s bottom line. By eliminating unnecessary complexity and distractions, businesses can:
- Improve operational efficiency and reduce costs
- Enhance their ability to innovate and adapt to changing market conditions
- Increase their focus on core competencies and high-priority initiatives
- Strengthen their competitive position and improve customer satisfaction
Implementing Strategic Subtraction
Implementing strategic subtraction requires careful planning, analysis, and execution. It involves:
- Identifying Non-Essential Elements: Conducting a thorough review of all business operations, products, and services to identify areas that do not align with the company’s strategic objectives or that are not generating sufficient value.
- Assessing Impact: Evaluating the potential impact of subtracting identified elements, including financial, operational, and customer implications.
- Developing a Subtraction Strategy: Creating a detailed plan for how subtraction will be implemented, including timelines, resource allocation, and communication strategies.
- Executing the Plan: Carrying out the subtraction strategy, which may involve restructuring, divestitures, or process simplification.
Overcoming Challenges
While strategic subtraction can be a powerful tool for improving business performance, it also presents several challenges. These can include:
- Resistance to Change: Employees and stakeholders may resist changes, especially if they involve job losses or significant alterations to business operations.
- Uncertainty and Risk: There is always a risk that subtracting certain elements could have unintended consequences, such as losing key customers or damaging the company’s reputation.
- Cultural Impact: Strategic subtraction can affect a company’s culture, potentially leading to demotivation or a loss of trust among employees if not managed carefully.
Case Studies and Examples
Several companies have successfully applied strategic subtraction to improve their performance. For example, a retail chain might decide to close underperforming stores to focus on more profitable locations. A technology firm might simplify its product lineup to concentrate on a fewer number of high-margin products. These actions can lead to significant improvements in efficiency, profitability, and competitiveness.
Best Practices for Strategic Subtraction
To maximize the benefits of strategic subtraction, companies should follow best practices such as:
- Engaging in thorough analysis and planning
- Communicating clearly with stakeholders
- Focusing on core competencies and high-value activities
- Monitoring and adjusting the subtraction strategy as needed
Conclusion
Strategic subtraction is a valuable approach for businesses looking to navigate turbulent times by simplifying operations, reducing costs, and enhancing focus on core objectives. By understanding the principles of strategic subtraction, identifying areas for improvement, and carefully planning and executing subtraction strategies, companies can position themselves for greater success and resilience in an ever-changing business environment.
FAQs
- Q: What is the primary goal of strategic subtraction?
A: The primary goal is to improve a company’s performance and resilience by eliminating non-essential elements. - Q: How do I identify what to subtract from my business?
A: Conduct a thorough review of your operations, products, and services to identify areas that do not align with your strategic objectives or generate insufficient value. - Q: What are the potential risks of strategic subtraction?
A: Risks include resistance to change, uncertainty, potential unintended consequences, and cultural impact. - Q: Can strategic subtraction apply to any business?
A: Yes, the principles of strategic subtraction can be applied to businesses of all sizes and industries, though the specific approaches may vary.
-
Career Advice6 months ago
Interview with Dr. Kristy K. Taylor, WORxK Global News Magazine Founder
-
Diversity and Inclusion (DEIA)6 months ago
Sarah Herrlinger Talks AirPods Pro Hearing Aid
-
Career Advice6 months ago
NetWork Your Way to Success: Top Tips for Maximizing Your Professional Network
-
Changemaker Interviews5 months ago
Unlocking Human Potential: Kim Groshek’s Journey to Transforming Leadership and Stress Resilience
-
Diversity and Inclusion (DEIA)6 months ago
The Power of Belonging: Why Feeling Accepted Matters in the Workplace
-
Global Trends and Politics6 months ago
Health-care stocks fall after Warren PBM bill, Brian Thompson shooting
-
Global Trends and Politics6 months ago
Unionization Goes Mainstream: How the Changing Workforce is Driving Demand for Collective Bargaining
-
Training and Development6 months ago
Level Up: How Upskilling Can Help You Stay Ahead of the Curve in a Rapidly Changing Industry